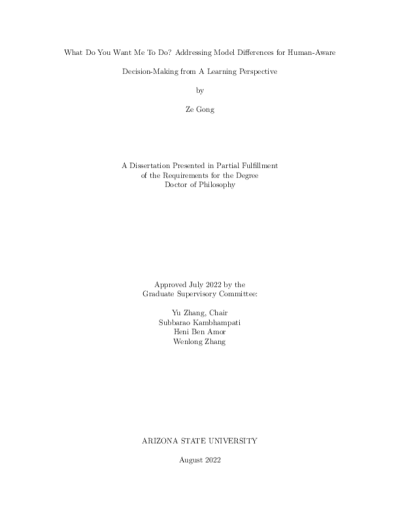
Description
As intelligent agents become pervasive in our lives, they are expected to not only achieve tasks alone but also engage in tasks with humans in the loop. In such cases, the human naturally forms an understanding of the agent, which affects his perception of the agent’s behavior. However, such an understanding inevitably deviates from the ground truth due to reasons such as the human’s lack of understanding of the domain or misunderstanding of the agent’s capabilities. Such differences would result in an unmatched expectation of the agent’s behavior with the agent’s optimal behavior, thereby biasing the human’s assessment of the agent’s performance. In this dissertation, I focus on when these differences are due to a biased belief about domain dynamics. I especially investigate the impact of such a biased belief on the agent’s decision-making process in two different problem settings from a learning perspective. In the first setting, the agent is tasked to accomplish a task alone but must infer the human’s objectives from the human’s feedback on the agent’s behavior in the environment. In such a case, the human biased feedback could mislead the agent to learn a reward function that results in a sub-optimal and, potentially, undesired policy. In the second setting, the agent must accomplish a task with a human observer. Given that the agent’s optimal behavior may not match the human’s expectation due to the biased belief, the agent’s optimal behavior may be viewed as inexplicable, leading to degraded performance and loss of trust. Consequently, this dissertation proposes approaches that (1) endow the agent with the ability to be aware of the human’s biased belief while inferring the human’s objectives, thereby (2) neutralize the impact of the model differences in a reinforcement learning framework, and (3) behave explicably by reconciling the human’s expectation and optimality during decision-making.
Download count: 2
Details
Title
- What Do You Want Me To Do? Addressing Model Differences for Human-Aware Decision-Making from A Learning Perspective
Contributors
- Gong, Ze (Author)
- Zhang, Yu (Thesis advisor)
- Amor, Hani Ben (Committee member)
- Kambhampati, Subbarao (Committee member)
- Zhang, Wenlong (Committee member)
- Arizona State University (Publisher)
Date Created
The date the item was original created (prior to any relationship with the ASU Digital Repositories.)
2022
Subjects
Resource Type
Collections this item is in
Note
-
Partial requirement for: Ph.D., Arizona State University, 2022
-
Field of study: Computer Science