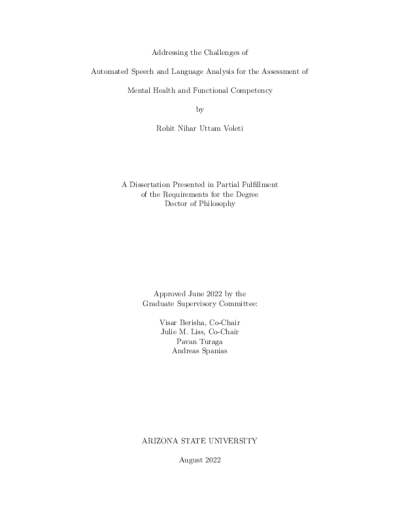
Description
Severe forms of mental illness, such as schizophrenia and bipolar disorder, are debilitating conditions that negatively impact an individual's quality of life. Additionally, they are often difficult and expensive to diagnose and manage, placing a large burden on society. Mental illness is typically diagnosed by the use of clinical interviews and a set of neuropsychiatric batteries; a key component of nearly all of these evaluations is some spoken language task. Clinicians have long used speech and language production as a proxy for neurological health, but most of these assessments are subjective in nature. Meanwhile, technological advancements in speech and natural language processing have grown exponentially over the past decade, increasing the capacity of computer models to assess particular aspects of speech and language. For this reason, many have seen an opportunity to leverage signal processing and machine learning applications to objectively assess clinical speech samples in order to automatically compute objective measures of neurological health. This document summarizes several contributions to expand upon this body of research. Mainly, there is still a large gap between the theoretical power of computational language models and their actual use in clinical applications. One of the largest concerns is the limited and inconsistent reliability of speech and language features used in models for assessing specific aspects of mental health; numerous methods may exist to measure the same or similar constructs and lead researchers to different conclusions in different studies. To address this, a novel measurement model based on a theoretical framework of speech production is used to motivate feature selection, while also performing a smoothing operation on features across several domains of interest. Then, these composite features are used to perform a much wider range of analyses than is typical of previous studies, looking at everything from diagnosis to functional competency assessments. Lastly, potential improvements to address practical implementation challenges associated with the use of speech and language technology in a real-world environment are investigated. The goal of this work is to demonstrate the ability of speech and language technology to aid clinical practitioners toward improvements in quality of life outcomes for their patients.
Details
Title
- Addressing the Challenges of Automated Speech and Language Analysis for the Assessment of Mental Health and Functional Competency
Contributors
- Voleti, Rohit Nihar Uttam (Author)
- Berisha, Visar (Thesis advisor)
- Liss, Julie M (Thesis advisor)
- Turaga, Pavan (Committee member)
- Spanias, Andreas (Committee member)
- Arizona State University (Publisher)
Date Created
The date the item was original created (prior to any relationship with the ASU Digital Repositories.)
2022
Resource Type
Collections this item is in
Note
-
Partial requirement for: Ph.D., Arizona State University, 2022
-
Field of study: Electrical Engineering