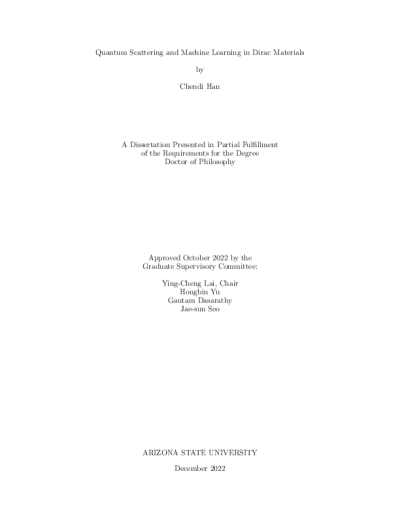
Description
A remarkable phenomenon in contemporary physics is quantum scarring in classically chaoticsystems, where the wave functions tend to concentrate on classical periodic orbits. Quantum
scarring has been studied for more than four decades, but the problem of efficiently detecting
quantum scars has remained to be challenging, relying mostly on human visualization of
wave function patterns. This paper develops a machine learning approach to detecting
quantum scars in an automated and highly efficient manner. In particular, this paper exploits Meta
learning. The first step is to construct a few-shot classification algorithm, under the
requirement that the one-shot classification accuracy be larger than 90%. Then propose a
scheme based on a combination of neural networks to improve the accuracy. This paper shows that
the machine learning scheme can find the correct quantum scars from thousands images of
wave functions, without any human intervention, regardless of the symmetry of the underlying
classical system. This will be the first application of Meta learning to quantum systems. Interacting spin networks are fundamental to quantum computing. Data-based tomography oftime-independent spin networks has been achieved, but an open challenge is to ascertain the
structures of time-dependent spin networks using time series measurements taken locally
from a small subset of the spins. Physically, the dynamical evolution of a spin network under
time-dependent driving or perturbation is described by the Heisenberg equation of motion.
Motivated by this basic fact, this paper articulates a physics-enhanced machine learning framework
whose core is Heisenberg neural networks. This paper demonstrates that, from local measurements, not only the local Hamiltonian can be recovered but the Hamiltonian reflecting the interacting structure of the whole system can
also be faithfully reconstructed. Using Heisenberg neural machine on spin networks of a
variety of structures. In the extreme case where measurements are taken from only one spin,
the achieved tomography fidelity values can reach about 90%. The developed machine
learning framework is applicable to any time-dependent systems whose quantum dynamical
evolution is governed by the Heisenberg equation of motion.
Details
Title
- Quantum Scattering and Machine Learning in Dirac Materials
Contributors
- Han, Chendi (Author)
- Lai, Ying-Cheng (Thesis advisor)
- Yu, Hongbin (Committee member)
- Dasarathy, Gautam (Committee member)
- Seo, Jae-Sun (Committee member)
- Arizona State University (Publisher)
Date Created
The date the item was original created (prior to any relationship with the ASU Digital Repositories.)
2022
Subjects
Resource Type
Collections this item is in
Note
- Partial requirement for: Ph.D., Arizona State University, 2022
- Field of study: Electrical Engineering