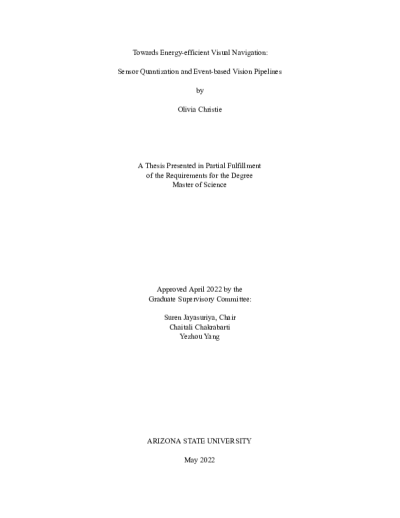
Description
Visual navigation is a useful and important task for a variety of applications. As the prevalence of robots increase, there is an increasing need for energy-efficient navigation methods as well. Many aspects of efficient visual navigation algorithms have been implemented in the literature, but there is a lack of work on evaluation of the efficiency of the image sensors. In this thesis, two methods are evaluated: adaptive image sensor quantization for traditional camera pipelines as well as new event-based sensors for low-power computer vision.The first contribution in this thesis is an evaluation of performing varying levels of sensor linear and logarithmic quantization with the task of visual simultaneous localization and mapping (SLAM). This unconventional method can provide efficiency benefits with a trade off between accuracy of the task and energy-efficiency. A new sensor quantization method, gradient-based quantization, is introduced to improve the accuracy of the task. This method only lowers the bit level of parts of the image that are less likely to be important in the SLAM algorithm since lower bit levels signify better energy-efficiency, but worse task accuracy. The third contribution is an evaluation of the efficiency and accuracy of event-based camera intensity representations for the task of optical flow. The results of performing a learning based optical flow are provided for each of five different reconstruction methods along with ablation studies. Lastly, the challenges of an event feature-based SLAM system are presented with results demonstrating the necessity for high quality and high resolution event data. The work in this thesis provides studies useful for examining tradeoffs for an efficient visual navigation system with traditional and event vision sensors. The results of this thesis also provide multiple directions for future work.
Details
Title
- Towards Energy-efficient Visual Navigation: Sensor Quantization and Event-based Vision Pipelines
Contributors
- Christie, Olivia Catherine (Author)
- Jayasuriya, Suren (Thesis advisor)
- Chakrabarti, Chaitali (Committee member)
- Yang, Yezhou (Committee member)
- Arizona State University (Publisher)
Date Created
The date the item was original created (prior to any relationship with the ASU Digital Repositories.)
2022
Subjects
Resource Type
Collections this item is in
Note
-
Partial requirement for: M.S., Arizona State University, 2022
-
Field of study: Engineering