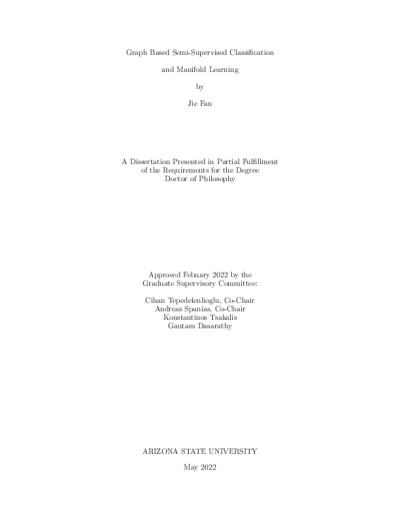
Description
Due to their effectiveness in capturing similarities between different entities, graphical models are widely used to represent datasets that reside on irregular and complex manifolds. Graph signal processing offers support to handle such complex datasets. By extending the digital signal processing conceptual frame from time and frequency domain to graph domain, operators such as graph shift, graph filter and graph Fourier transform are defined. In this dissertation, two novel graph filter design methods are proposed. First, a graph filter with multiple shift matrices is applied to semi-supervised classification, which can handle features with uneven qualities through an embedded feature importance evaluation process. Three optimization solutions are provided: an alternating minimization method that is simple to implement, a convex relaxation method that provides a theoretical performance benchmark and a genetic algorithm, which is computationally efficient and better at configuring overfitting. Second, a graph filter with splitting-and-merging scheme is proposed, which splits the graph into multiple subgraphs. The corresponding subgraph filters are trained parallelly and in the last, by merging all the subgraph filters, the final graph filter is obtained. Due to the splitting process, the redundant edges in the original graph are dropped, which can save computational cost in semi-supervised classification. At the same time, this scheme also enables the filter to represent unevenly sampled data in manifold learning. To evaluate the performance of the proposed graph filter design approaches, simulation experiments with synthetic and real datasets are conduct. The Monte Carlo cross validation method is employed to demonstrate the need for the proposed graph filter design approaches in various application scenarios. Criterions, such as accuracy, Gini score, F1-score and learning curves, are provided to analyze the performance of the proposed methods and their competitors.
Download count: 2
Details
Title
- Graph Based Semi-Supervised Classification and Manifold Learning
Contributors
- Fan, Jie (Author)
- Tepedelenlioğlu, Cihan (Thesis advisor)
- Spanias, Andreas (Thesis advisor)
- Tsakalis, Konstantinos (Committee member)
- Dasarathy, Gautam (Committee member)
- Arizona State University (Publisher)
Date Created
The date the item was original created (prior to any relationship with the ASU Digital Repositories.)
2022
Subjects
Resource Type
Collections this item is in
Note
-
Partial requirement for: Ph.D., Arizona State University, 2022
-
Field of study: Electrical Engineering