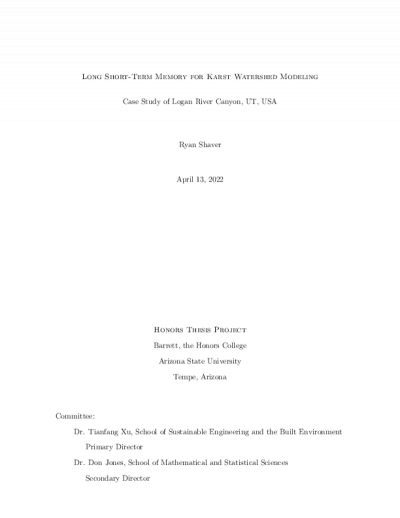
Description
Hydrologic modeling in snowfed karst watersheds is important for many communities relying on their water for municipal and agricultural use, but the complexities of karst hydrology have made this task historically difficult. Here, two Long Short-Term Memory (LSTM) models are compared to investigate this problem from a deep-learning perspective within the context of the Logan River Canyon watershed, which supplies water to Logan City, UT. One is spatially lumped and the other spatially distributed, the latter with a potential to reveal underlying spatial watershed dynamics. Both use snowmelt and rainfall to predict daily streamflow downstream. I find distributed LSTMs consistently outperform lumped LSTMs in this task. Additionally, I find that a spatial sensitivity analysis of distributed LSTMs is unpromising in revealing spatial watershed dynamics but warrants further investigation.
Details
Title
- Long Short-Term Memory for Karst Watershed Modeling: Case Study of Logan River Canyon, UT, USA
Contributors
- Shaver, Ryan (Author)
- Xu, Tianfang (Thesis director)
- Jones, Don (Committee member)
- Barrett, The Honors College (Contributor)
- School of Earth and Space Exploration (Contributor)
- School of Mathematical and Statistical Sciences (Contributor)
Date Created
The date the item was original created (prior to any relationship with the ASU Digital Repositories.)
2022-05
Subjects
Resource Type
Collections this item is in