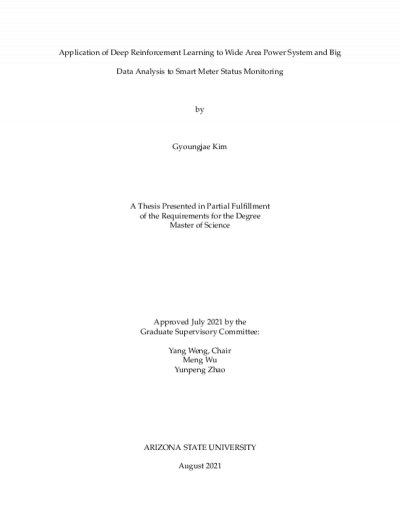
Description
Due to the large scale of power systems, latency uncertainty in communication can cause severe problems in wide-area measurement systems. To resolve the issue, a significant amount of past work focuses on using emerging technologywhich is machine learning methods such as Q-learning to address latency issues in modern controls. Although such a method can deal with the stochastic characteristics of communication latency in the long run, the Q-learning methods tend to overestimate Q-values, leading to high bias. To solve the overestimation bias issue, the learning structure is redesigned with a twin-delayed deep deterministic policy gradient algorithm to handle the damping control issue under unknown latency in the power network. Meanwhile, a new reward function is proposed, taking into account the machine speed deviation, the episode termination prevention, and the feedback from action space. In this way, the system optimally damps down frequency oscillations while maintaining the system’s stability and reliable operation within defined limits. The simulation results verify the proposed algorithm in various perspectives including the latency sensitivity analysis under high renewable energy penetration and the comparison with other machine learning algorithms.
For example, if the proposed twin-delayed deep deterministic policy gradient algorithm is applied, the low-frequency oscillation significantly improved compared to existing algorithms.
Furthermore, under the mentorship of Dr. Yang Weng, the development of a big data analysis software project has been collaborating with the Salt River Project (SRP), a major power utility in Arizona. After a thorough examination of data for
the project, it is examined that SRP is suffering from a lot of smart meters data issues. An important goal of the project is to design big data software to monitor SRP smart meter data and to present indicators of abnormalities and special
events. Currently, the big data software interface has been developed for SRP, which has already been successfully adopted by other utilities, research institutes, and laboratories as well.
Download count: 3
Details
Title
- Application of Deep Reinforcement Learning to Wide Area Power System and Big Data Analysis to Smart Meter Status Monitoring
Contributors
- Kim, Gyoungjae (Author)
- Weng, Yang (Thesis advisor)
- Wu, Meng (Committee member)
- Zhao, Yunpeng (Committee member)
- Arizona State University (Publisher)
Date Created
The date the item was original created (prior to any relationship with the ASU Digital Repositories.)
2021
Resource Type
Collections this item is in
Note
-
Partial requirement for: M.S., Arizona State University, 2021
-
Field of study: Electrical Engineering