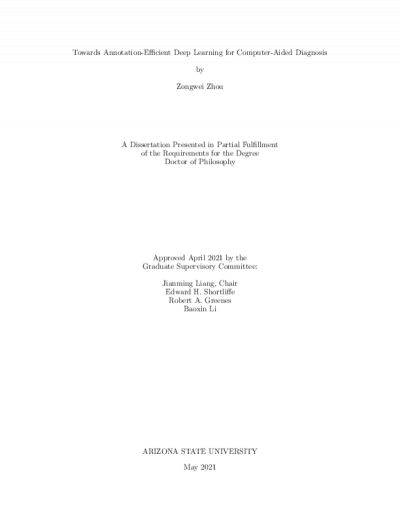
Description
There is intense interest in adopting computer-aided diagnosis (CAD) systems, particularly those developed based on deep learning algorithms, for applications in a number of medical specialties. However, success of these CAD systems relies heavily on large annotated datasets; otherwise, deep learning often results in algorithms that perform poorly and lack generalizability. Therefore, this dissertation seeks to address this critical problem: How to develop efficient and effective deep learning algorithms for medical applications where large annotated datasets are unavailable. In doing so, we have outlined three specific aims: (1) acquiring necessary annotations efficiently from human experts; (2) utilizing existing annotations effectively from advanced architecture; and (3) extracting generic knowledge directly from unannotated images. Our extensive experiments indicate that, with a small part of the dataset annotated, the developed deep learning methods can match, or even outperform those that require annotating the entire dataset. The last part of this dissertation presents the importance and application of imaging in healthcare, elaborating on how the developed techniques can impact several key facets of the CAD system for detecting pulmonary embolism. Further research is necessary to determine the feasibility of applying these advanced deep learning technologies in clinical practice, particularly when annotation is limited. Progress in this area has the potential to enable deep learning algorithms to generalize to real clinical data and eventually allow CAD systems to be employed in clinical medicine at the point of care.
Details
Title
- Towards Annotation-Efficient Deep Learning for Computer-Aided Diagnosis
Contributors
- Zhou, Zongwei (Author)
- Liang, Jianming (Thesis advisor)
- Shortliffe, Edward H (Committee member)
- Greenes, Robert A (Committee member)
- Li, Baoxin (Committee member)
- Arizona State University (Publisher)
Date Created
The date the item was original created (prior to any relationship with the ASU Digital Repositories.)
2021
Subjects
Resource Type
Collections this item is in
Note
-
Partial requirement for: Ph.D., Arizona State University, 2021
-
Field of study: Biomedical Informatics