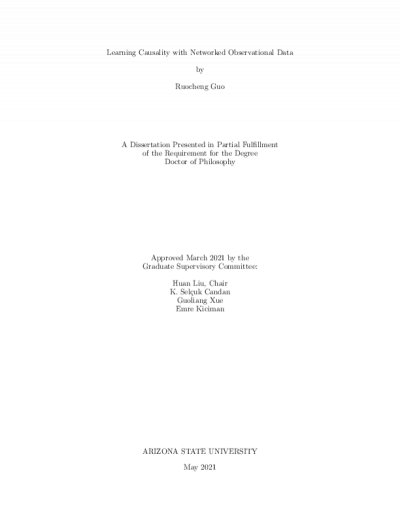
Description
This dissertation considers the question of how convenient access to copious networked observational data impacts our ability to learn causal knowledge. It investigates in what ways learning causality from such data is different from -- or the same as -- the traditional causal inference which often deals with small scale i.i.d. data collected from randomized controlled trials? For example, how can we exploit network information for a series of tasks in the area of learning causality? To answer this question, the dissertation is written toward developing a suite of novel causal learning algorithms that offer actionable insights for a series of causal inference tasks with networked observational data. The work aims to benefit real-world decision-making across a variety of highly influential applications. In the first part of this dissertation, it investigates the task of inferring individual-level causal effects from networked observational data. First, it presents a representation balancing-based framework for handling the influence of hidden confounders to achieve accurate estimates of causal effects. Second, it extends the framework with an adversarial learning approach to properly combine two types of existing heuristics: representation balancing and treatment prediction. The second part of the dissertation describes a framework for counterfactual evaluation of treatment assignment policies with networked observational data. A novel framework that captures patterns of hidden confounders is developed to provide more informative input for downstream counterfactual evaluation methods. The third part presents a framework for debiasing two-dimensional grid-based e-commerce search with observational search log data where there is an implicit network connecting neighboring products in a search result page. A novel inverse propensity scoring framework that models user behavior patterns for two-dimensional display in e-commerce websites is developed, which aims to optimize online performance of ranking algorithms with offline log data.
Download count: 2
Details
Title
- Learning Causality with Networked Observational Data
Contributors
- Guo, Ruocheng (Author)
- Liu, Huan (Thesis advisor)
- Candan, K. Selcuk (Committee member)
- Xue, Guoliang (Committee member)
- Kiciman, Emre (Committee member)
- Arizona State University (Publisher)
Date Created
The date the item was original created (prior to any relationship with the ASU Digital Repositories.)
2021
Subjects
Resource Type
Collections this item is in
Note
-
Partial requirement for: Ph.D., Arizona State University, 2021
-
Field of study: Computer Engineering