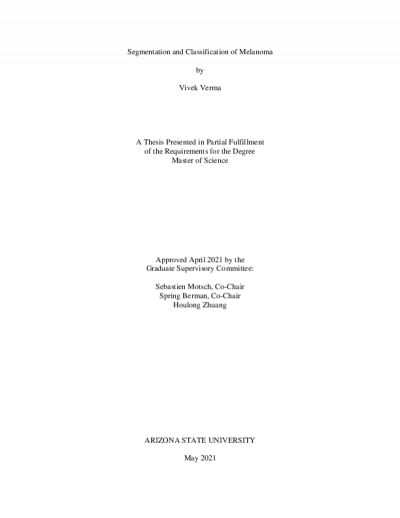
Description
A skin lesion is a part of the skin which has an uncommon growth or appearance in comparison with the skin around it. While most are harmless, some can be warnings of skin cancer. Melanoma is the deadliest form of skin cancer and its early detection in dermoscopic images is crucial and results in increase in the survival rate. The clinical ABCD (asymmetry, border irregularity, color variation and diameter greater than 6mm) rule is one of the most widely used method for early melanoma recognition. However, accurate classification of melanoma is still extremely difficult due to following reasons(not limited to): great visual resemblance between melanoma and non-melanoma skin lesions, less contrast difference between skin and the lesions etc. There is an ever-growing need of correct and reliable detection of skin cancers. Advances in the field of deep learning deems it perfect for the task of automatic detection and is very useful to pathologists as they aid them in terms of efficiency and accuracy. In this thesis various state of the art deep learning frameworks are used. An analysis of their parameters is done, innovative techniques are implemented to address the challenges faced in the tasks, segmentation, and classification in skin lesions.• Segmentation is task of dividing out regions of interest. This is used to only keep the ROI and separate it from its background.
• Classification is the task of assigning the image a class, i.e., Melanoma(Cancer) and Nevus(Not Cancer). A pre-trained model is used and fine-tuned as per the needs of the given problem statement/dataset.
Experimental results show promise as the implemented techniques reduce the false negatives rate, i.e., neural network is less likely to misclassify a melanoma.
Download count: 1
Details
Title
- Segmentation and Classification of Melanoma
Contributors
- Verma, Vivek (Author)
- Motsch, Sebastien (Thesis advisor)
- Berman, Spring (Thesis advisor)
- Zhuang, Houlong (Committee member)
- Arizona State University (Publisher)
Date Created
The date the item was original created (prior to any relationship with the ASU Digital Repositories.)
2021
Subjects
Resource Type
Collections this item is in
Note
-
Partial requirement for: M.S., Arizona State University, 2021
-
Field of study: Mechanical Engineering