Description
Standardization is sorely lacking in the field of musical machine learning. This thesis project endeavors to contribute to this standardization by training three machine learning models on the same dataset and comparing them using the same metrics. The music-specific metrics utilized provide more relevant information for diagnosing the shortcomings of each model.
Included in this item (2)
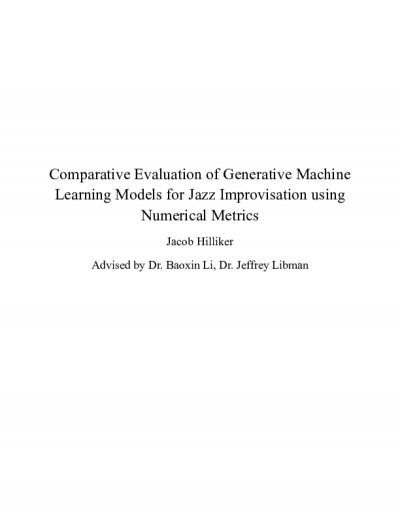
Permanent Link
Contributors
Hilliker, Jacob (Author) / Li, Baoxin (Thesis director) / Libman, Jeffrey (Committee member) / Barrett, The Honors College (Contributor) / Computer Science and Engineering Program (Contributor)
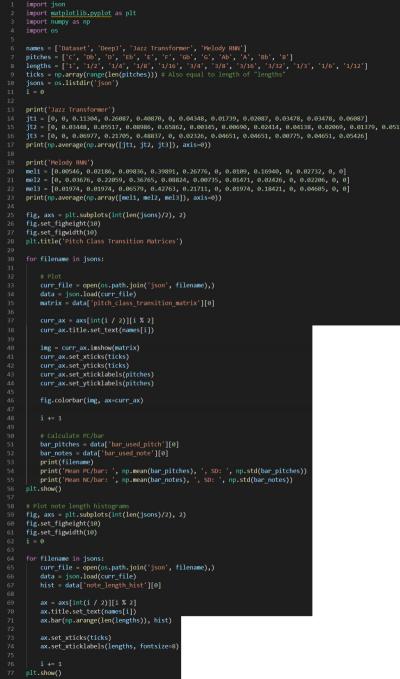
Permanent Link
Contributors
Hilliker, Jacob (Author) / Li, Baoxin (Thesis director) / Libman, Jeffrey (Committee member) / Barrett, The Honors College (Contributor) / Computer Science and Engineering Program (Contributor)
Details
Title
- Comparative Evaluation of Generative Machine Learning Models for Jazz Improvisation using Numerical Metrics
Contributors
Agent
- Hilliker, Jacob (Author)
- Li, Baoxin (Thesis director)
- Libman, Jeffrey (Committee member)
- Barrett, The Honors College (Contributor)
- Computer Science and Engineering Program (Contributor)
Date Created
The date the item was original created (prior to any relationship with the ASU Digital Repositories.)
2021-12
Subjects
Collections this item is in