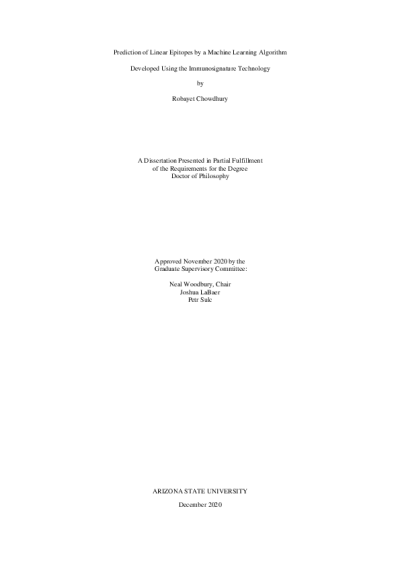
Description
Elucidation of Antigen-Antibody (Ag-Ab) interactions is critical to the understanding of humoral immune responses to pathogenic infection. B cells are crucial components of the immune system that generate highly specific antibodies, such as IgG, towards epitopes on antigens. Serum IgG molecules carry specific molecular recognition information concerning the antigens that initiated their production. If one could read it, this information can be used to predict B cell epitopes on target antigens in order to design effective epitope driven vaccines, therapies and serological assays. Immunosignature technology captures the specific information content of serum IgG from infected and uninfected individuals on high density microarrays containing ~105 nearly random peptide sequences. Although the sequences of the peptides are chosen to evenly cover amino acid sequence space, the pattern of serum IgG binding to the array contains a consistent signature associated with each specific disease (e.g., Valley fever, influenza) among many individuals. Here, the disease specific but agnostic behavior of the technology has been explored by profiling molecular recognition information for five pathogens causing life threatening infectious diseases (e.g. DENV, WNV, HCV, HBV, and T.cruzi). This was done by models developed using a machine learning algorithm to model the sequence dependence of the humoral immune responses as measured by the peptide arrays. It was shown that the disease specific binding information could be accurately related to the peptide sequences used on the array by the machine learning (ML) models. Importantly, it was demonstrated that the ML models could identify or predict known linear epitopes on antigens of the four viruses. Moreover, the models identified potential novel linear epitopes on antigens of the four viruses (each has 4-10 proteins in the proteome) and of T.cruzi (a eukaryotic parasite which has over 12,000 proteins in its proteome). Finally, the predicted epitopes were tested in serum IgG binding assays such as ELISAs. Unfortunately, the assay results were inconsistent due to problems with peptide/surface interactions. In a separate study for the development of antibody recruiting molecules (ARMs) to combat microbial infections, 10 peptides from the high density peptide arrays were tested in IgG binding assays using sera of healthy individuals to find a set of antibody binding termini (ABT, a ligand that binds to a variable region of the IgG). It was concluded that one peptide (peptide 7) may be used as a potential ABT. Overall, these findings demonstrate the applications of the immunosignature technology ranging from developing tools to predict linear epitopes on pathogens of small to large proteomes to the identification of an ABT for ARMs.
Details
Title
- Prediction of Linear Epitopes by a Machine Learning Algorithm Developed Using the Immunosignature Technology
Contributors
- CHOWDHURY, ROBAYET (Author)
- Woodbury, Neal (Thesis advisor)
- LaBaer, Joshua (Committee member)
- Sulc, Petr (Committee member)
- Arizona State University (Publisher)
Date Created
The date the item was original created (prior to any relationship with the ASU Digital Repositories.)
2020
Subjects
Resource Type
Collections this item is in
Note
- Doctoral Dissertation Biochemistry 2020