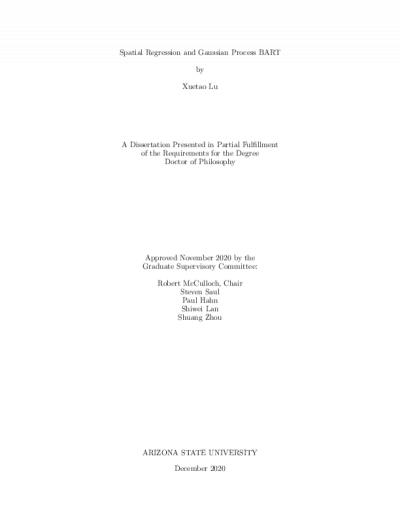
Description
Spatial regression is one of the central topics in spatial statistics. Based on the goals, interpretation or prediction, spatial regression models can be classified into two categories, linear mixed regression models and nonlinear regression models. This dissertation explored these models and their real world applications. New methods and models were proposed to overcome the challenges in practice. There are three major parts in the dissertation.
In the first part, nonlinear regression models were embedded into a multistage workflow to predict the spatial abundance of reef fish species in the Gulf of Mexico. There were two challenges, zero-inflated data and out of sample prediction. The methods and models in the workflow could effectively handle the zero-inflated sampling data without strong assumptions. Three strategies were proposed to solve the out of sample prediction problem. The results and discussions showed that the nonlinear prediction had the advantages of high accuracy, low bias and well-performed in multi-resolution.
In the second part, a two-stage spatial regression model was proposed for analyzing soil carbon stock (SOC) data. In the first stage, there was a spatial linear mixed model that captured the linear and stationary effects. In the second stage, a generalized additive model was used to explain the nonlinear and nonstationary effects. The results illustrated that the two-stage model had good interpretability in understanding the effect of covariates, meanwhile, it kept high prediction accuracy which is competitive to the popular machine learning models, like, random forest, xgboost and support vector machine.
A new nonlinear regression model, Gaussian process BART (Bayesian additive regression tree), was proposed in the third part. Combining advantages in both BART and Gaussian process, the model could capture the nonlinear effects of both observed and latent covariates. To develop the model, first, the traditional BART was generalized to accommodate correlated errors. Then, the failure of likelihood based Markov chain Monte Carlo (MCMC) in parameter estimating was discussed. Based on the idea of analysis of variation, back comparing and tuning range, were proposed to tackle this failure. Finally, effectiveness of the new model was examined by experiments on both simulation and real data.
In the first part, nonlinear regression models were embedded into a multistage workflow to predict the spatial abundance of reef fish species in the Gulf of Mexico. There were two challenges, zero-inflated data and out of sample prediction. The methods and models in the workflow could effectively handle the zero-inflated sampling data without strong assumptions. Three strategies were proposed to solve the out of sample prediction problem. The results and discussions showed that the nonlinear prediction had the advantages of high accuracy, low bias and well-performed in multi-resolution.
In the second part, a two-stage spatial regression model was proposed for analyzing soil carbon stock (SOC) data. In the first stage, there was a spatial linear mixed model that captured the linear and stationary effects. In the second stage, a generalized additive model was used to explain the nonlinear and nonstationary effects. The results illustrated that the two-stage model had good interpretability in understanding the effect of covariates, meanwhile, it kept high prediction accuracy which is competitive to the popular machine learning models, like, random forest, xgboost and support vector machine.
A new nonlinear regression model, Gaussian process BART (Bayesian additive regression tree), was proposed in the third part. Combining advantages in both BART and Gaussian process, the model could capture the nonlinear effects of both observed and latent covariates. To develop the model, first, the traditional BART was generalized to accommodate correlated errors. Then, the failure of likelihood based Markov chain Monte Carlo (MCMC) in parameter estimating was discussed. Based on the idea of analysis of variation, back comparing and tuning range, were proposed to tackle this failure. Finally, effectiveness of the new model was examined by experiments on both simulation and real data.
Details
Title
- Spatial Regression and Gaussian Process BART
Contributors
- Lu, Xuetao (Author)
- McCulloch, Robert (Thesis advisor)
- Hahn, Paul (Committee member)
- Lan, Shiwei (Committee member)
- Zhou, Shuang (Committee member)
- Saul, Steven (Committee member)
- Arizona State University (Publisher)
Date Created
The date the item was original created (prior to any relationship with the ASU Digital Repositories.)
2020
Subjects
Resource Type
Collections this item is in
Note
- Doctoral Dissertation Statistics 2020