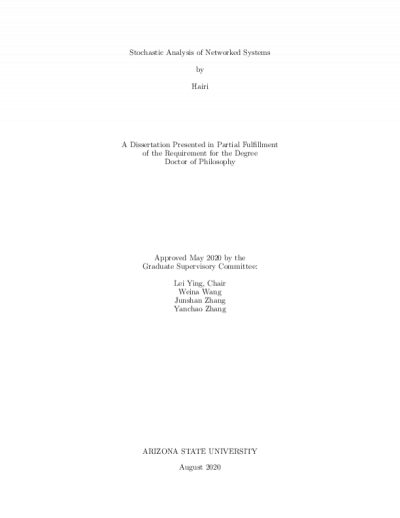
Description
This dissertation presents a novel algorithm for recovering missing values of co-evolving time series with partial embedded network information. The idea is to connect two sources of data through a shared low dimensional latent space. The proposed algorithm, named NetDyna, is an Expectation-Maximization algorithm, and uses the Kalman filter and matrix factorization approaches to infer the missing values both in the time series and embedded network. The experimental results on real datasets, including a Motes dataset and a Motion Capture dataset, show that (1) NetDyna outperforms other state-of-the-art algorithms, especially with partially observed network information; (2) its computational complexity scales linearly with the time duration of time series; and (3) the algorithm recovers the embedded network in addition to missing time series values.
This dissertation also studies a load balancing algorithm, the so called power-of-two-choices(Po2), for many-server systems (with N servers) and focuses on the convergence of stationary distribution of Po2 in the both light and heavy traffic regimes to the solution of mean-field system. The framework of Stein’s method and state space collapse (SSC) are used to analyze both regimes.
In both regimes, the thesis first uses the argument of state space collapse to show that the probability of the state being far from the mean-field solution is small enough. By a simple Markov inequality, it is able to show that the probability is indeed very small with a proper choice of parameters.
Then, for the state space close to the solution of mean-field model, the thesis uses Stein’s method to show that the stochastic system is close to a linear mean-field model. By characterizing the generator difference, it is able to characterize the dominant terms in both regimes. Note that for heavy traffic case, the lower and upper bound analysis of a tridiagonal matrix, which arises from the linear mean-field model, is needed. From the dominant term, it allows to calculate the coefficient of the convergence rate.
In the end, comparisons between the theoretical predictions and numerical simulations are presented.
This dissertation also studies a load balancing algorithm, the so called power-of-two-choices(Po2), for many-server systems (with N servers) and focuses on the convergence of stationary distribution of Po2 in the both light and heavy traffic regimes to the solution of mean-field system. The framework of Stein’s method and state space collapse (SSC) are used to analyze both regimes.
In both regimes, the thesis first uses the argument of state space collapse to show that the probability of the state being far from the mean-field solution is small enough. By a simple Markov inequality, it is able to show that the probability is indeed very small with a proper choice of parameters.
Then, for the state space close to the solution of mean-field model, the thesis uses Stein’s method to show that the stochastic system is close to a linear mean-field model. By characterizing the generator difference, it is able to characterize the dominant terms in both regimes. Note that for heavy traffic case, the lower and upper bound analysis of a tridiagonal matrix, which arises from the linear mean-field model, is needed. From the dominant term, it allows to calculate the coefficient of the convergence rate.
In the end, comparisons between the theoretical predictions and numerical simulations are presented.
Download count: 1
Details
Title
- Stochastic Analysis of Networked Systems
Contributors
- Hairi, FNU (Author)
- Ying, Lei (Thesis advisor)
- Wang, Weina (Committee member)
- Zhang, Junshan (Committee member)
- Zhang, Yanchao (Committee member)
- Arizona State University (Publisher)
Date Created
The date the item was original created (prior to any relationship with the ASU Digital Repositories.)
2020
Subjects
Resource Type
Collections this item is in
Note
-
Doctoral Dissertation Electrical Engineering 2020