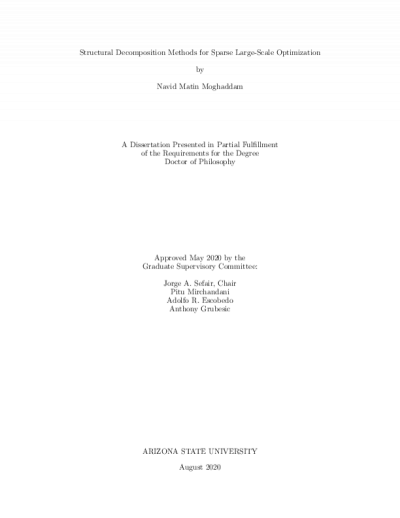
Description
This dissertation focuses on three large-scale optimization problems and devising algorithms to solve them. In addition to the societal impact of each problem’s solution, this dissertation contributes to the optimization literature a set of decomposition algorithms for problems whose optimal solution is sparse. These algorithms exploit problem-specific properties and use tailored strategies based on iterative refinement (outer-approximations). The proposed algorithms are not rooted in duality theory, providing an alternative to existing methods based on linear programming relaxations. However, it is possible to embed existing decomposition methods into the proposed framework. These general decomposition principles extend to other combinatorial optimization problems.
The first problem is a route assignment and scheduling problem in which a set of vehicles need to traverse a directed network while maintaining a minimum inter-vehicle distance at any time. This problem is inspired by applications in hazmat logistics and the coordination of autonomous agents. The proposed approach includes realistic features such as continuous-time vehicle scheduling, heterogeneous speeds, minimum and maximum waiting times at any node, among others.
The second problem is a fixed-charge network design, which aims to find a minimum-cost plan to transport a target amount of a commodity between known origins and destinations. In addition to the typical flow decisions, the model chooses the capacity of each arc and selects sources and sinks. The proposed algorithms admit any nondecreasing piecewise linear cost structure. This model is applied to the Carbon Capture and Storage (CCS) problem, which is to design a minimum-cost pipeline network to transport CO2 between industrial sources and geologic reservoirs for long-term storage.
The third problem extends the proposed decomposition framework to a special case of joint chance constraint programming with independent random variables. This model is applied to the probabilistic transportation problem, where demands are assumed stochastic and independent. Using an empirical probability distribution, this problem is formulated as an integer program with the goal of finding a minimum-cost distribution plan that satisfies all the demands with a minimum given probability. The proposed scalable algorithm is based on a concave envelop approximation of the empirical probability function, which is iteratively refined as needed.
The first problem is a route assignment and scheduling problem in which a set of vehicles need to traverse a directed network while maintaining a minimum inter-vehicle distance at any time. This problem is inspired by applications in hazmat logistics and the coordination of autonomous agents. The proposed approach includes realistic features such as continuous-time vehicle scheduling, heterogeneous speeds, minimum and maximum waiting times at any node, among others.
The second problem is a fixed-charge network design, which aims to find a minimum-cost plan to transport a target amount of a commodity between known origins and destinations. In addition to the typical flow decisions, the model chooses the capacity of each arc and selects sources and sinks. The proposed algorithms admit any nondecreasing piecewise linear cost structure. This model is applied to the Carbon Capture and Storage (CCS) problem, which is to design a minimum-cost pipeline network to transport CO2 between industrial sources and geologic reservoirs for long-term storage.
The third problem extends the proposed decomposition framework to a special case of joint chance constraint programming with independent random variables. This model is applied to the probabilistic transportation problem, where demands are assumed stochastic and independent. Using an empirical probability distribution, this problem is formulated as an integer program with the goal of finding a minimum-cost distribution plan that satisfies all the demands with a minimum given probability. The proposed scalable algorithm is based on a concave envelop approximation of the empirical probability function, which is iteratively refined as needed.
Details
Title
- Structural Decomposition Methods for Sparse Large-Scale Optimization
Contributors
- Matin Moghaddam, Navid (Author)
- Sefair, Jorge (Thesis advisor)
- Mirchandani, Pitu (Committee member)
- Escobedo, Adolfo (Committee member)
- Grubesic, Anthony (Committee member)
- Arizona State University (Publisher)
Date Created
The date the item was original created (prior to any relationship with the ASU Digital Repositories.)
2020
Resource Type
Collections this item is in
Note
-
Doctoral Dissertation Industrial Engineering 2020