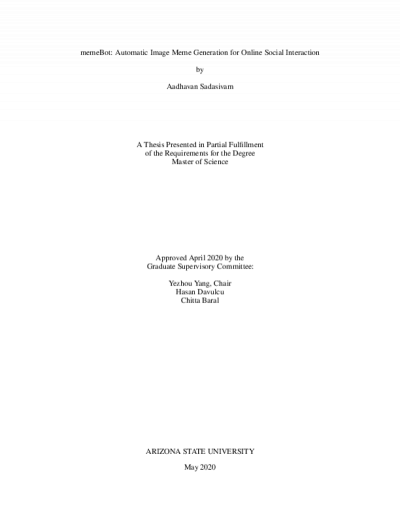
Description
Internet memes have become a widespread tool used by people for interacting and exchanging ideas over social media, blogs, and open messengers. Internet memes most commonly take the form of an image which is a combination of image, text, and humor, making them a powerful tool to deliver information. Image memes are used in viral marketing and mass advertising to propagate any ideas ranging from simple commercials to those that can cause changes and development in the social structures like countering hate speech.
This work proposes to treat automatic image meme generation as a translation process, and further present an end to end neural and probabilistic approach to generate an image-based meme for any given sentence using an encoder-decoder architecture. For a given input sentence, a meme is generated by combining a meme template image and a text caption where the meme template image is selected from a set of popular candidates using a selection module and the meme caption is generated by an encoder-decoder model. An encoder is used to map the selected meme template and the input sentence into a meme embedding space and then a decoder is used to decode the meme caption from the meme embedding space. The generated natural language caption is conditioned on the input sentence and the selected meme template.
The model learns the dependencies between the meme captions and the meme template images and generates new memes using the learned dependencies. The quality of the generated captions and the generated memes is evaluated through both automated metrics and human evaluation. An experiment is designed to score how well the generated memes can represent popular tweets from Twitter conversations. Experiments on Twitter data show the efficacy of the model in generating memes capable of representing a sentence in online social interaction.
This work proposes to treat automatic image meme generation as a translation process, and further present an end to end neural and probabilistic approach to generate an image-based meme for any given sentence using an encoder-decoder architecture. For a given input sentence, a meme is generated by combining a meme template image and a text caption where the meme template image is selected from a set of popular candidates using a selection module and the meme caption is generated by an encoder-decoder model. An encoder is used to map the selected meme template and the input sentence into a meme embedding space and then a decoder is used to decode the meme caption from the meme embedding space. The generated natural language caption is conditioned on the input sentence and the selected meme template.
The model learns the dependencies between the meme captions and the meme template images and generates new memes using the learned dependencies. The quality of the generated captions and the generated memes is evaluated through both automated metrics and human evaluation. An experiment is designed to score how well the generated memes can represent popular tweets from Twitter conversations. Experiments on Twitter data show the efficacy of the model in generating memes capable of representing a sentence in online social interaction.
Download count: 7
Details
Title
- memeBot: Automatic Image Meme Generation for Online Social Interaction
Contributors
- Sadasivam, Aadhavan (Author)
- Yang, Yezhou (Thesis advisor)
- Baral, Chitta (Committee member)
- Davulcu, Hasan (Committee member)
- Arizona State University (Publisher)
Date Created
The date the item was original created (prior to any relationship with the ASU Digital Repositories.)
2020
Subjects
Resource Type
Collections this item is in
Note
-
Masters Thesis Computer Science 2020