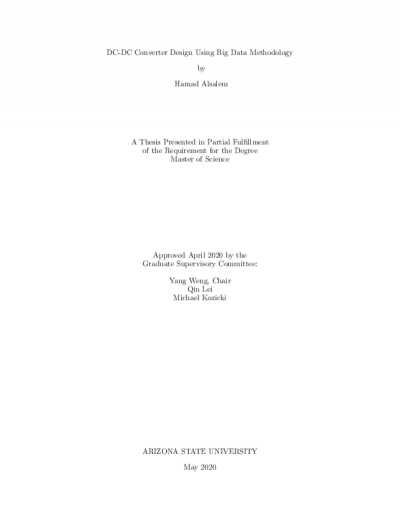
Description
With the rapid advancement in the technologies related to renewable energies such
as solar, wind, fuel cell, and many more, there is a definite need for new power con
verting methods involving data-driven methodology. Having adequate information is
crucial for any innovative ideas to fructify; accordingly, moving away from traditional
methodologies is the most practical way of giving birth to new ideas. While working
on a DC-DC buck converter, the input voltages considered for running the simulations
are varied for research purposes. The critical aspect of the new data-driven method
ology is to propose a machine learning algorithm. In this design, solving for inductor
value and power switching losses, the parameters can be achieved while keeping the
input and output ratio close to the value as necessary. Thus, implementing machine
learning algorithms with the traditional design of a non-isolated buck converter deter
mines the optimal outcome for the inductor value and power loss, which is achieved
by assimilating a DC-DC converter and data-driven methodology.
The present thesis investigates the different outcomes from machine learning al
gorithms in comparison with the dynamic equations. Specifically, the DC-DC buck
converter will be focused on the thesis. In order to determine the most effective way
of keeping the system in a steady-state, different circuit buck converter with different
parameters have been performed.
At present, artificial intelligence plays a vital role in power system control and
theory. Consequently, in this thesis, the approximation error estimation has been
analyzed in a DC-DC buck converter model, with specific consideration of machine
learning algorithms tools that can help detect and calculate the difference in terms
of error. These tools, called models, are used to analyze the collected data. In the
present thesis, a focus on such models as K-nearest neighbors (K-NN), specifically
the Weighted-nearest neighbor (WKNN), is utilized for machine learning algorithm
purposes. The machine learning concept introduced in the present thesis lays down
the foundation for future research in this area so that to enable further research on
efficient ways to improve power electronic devices with reduced power switching losses
and optimal inductor values.
as solar, wind, fuel cell, and many more, there is a definite need for new power con
verting methods involving data-driven methodology. Having adequate information is
crucial for any innovative ideas to fructify; accordingly, moving away from traditional
methodologies is the most practical way of giving birth to new ideas. While working
on a DC-DC buck converter, the input voltages considered for running the simulations
are varied for research purposes. The critical aspect of the new data-driven method
ology is to propose a machine learning algorithm. In this design, solving for inductor
value and power switching losses, the parameters can be achieved while keeping the
input and output ratio close to the value as necessary. Thus, implementing machine
learning algorithms with the traditional design of a non-isolated buck converter deter
mines the optimal outcome for the inductor value and power loss, which is achieved
by assimilating a DC-DC converter and data-driven methodology.
The present thesis investigates the different outcomes from machine learning al
gorithms in comparison with the dynamic equations. Specifically, the DC-DC buck
converter will be focused on the thesis. In order to determine the most effective way
of keeping the system in a steady-state, different circuit buck converter with different
parameters have been performed.
At present, artificial intelligence plays a vital role in power system control and
theory. Consequently, in this thesis, the approximation error estimation has been
analyzed in a DC-DC buck converter model, with specific consideration of machine
learning algorithms tools that can help detect and calculate the difference in terms
of error. These tools, called models, are used to analyze the collected data. In the
present thesis, a focus on such models as K-nearest neighbors (K-NN), specifically
the Weighted-nearest neighbor (WKNN), is utilized for machine learning algorithm
purposes. The machine learning concept introduced in the present thesis lays down
the foundation for future research in this area so that to enable further research on
efficient ways to improve power electronic devices with reduced power switching losses
and optimal inductor values.
Details
Title
- DC-DC Converter Design Using Big Data Methodology
Contributors
- Alsalem, Hamad (Author)
- Weng, Yang (Thesis advisor)
- Lei, Qin (Committee member)
- Kozicki, Michael (Committee member)
- Arizona State University (Publisher)
Date Created
The date the item was original created (prior to any relationship with the ASU Digital Repositories.)
2020
Subjects
Resource Type
Collections this item is in
Note
- Masters Thesis Electrical Engineering 2020