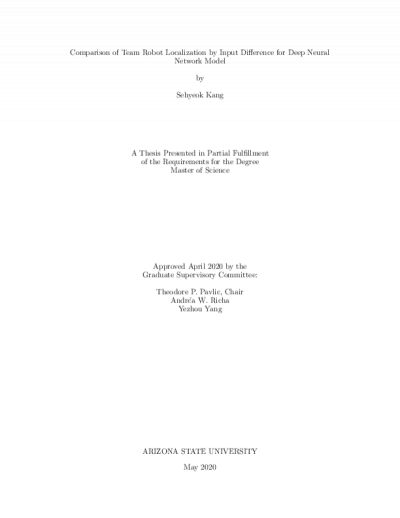
Description
In a multi-robot system, locating a team robot is an important issue. If robots
can refer to the location of team robots based on information through passive action
recognition without explicit communication, various advantages (e.g. improving security
for military purposes) can be obtained. Specifically, when team robots follow
the same motion rule based on information about adjacent robots, associations can
be found between robot actions. If the association can be analyzed, this can be a clue
to the remote robot. Using these clues, it is possible to infer remote robots which are
outside of the sensor range.
In this paper, a multi-robot system is constructed using a combination of Thymio
II robotic platforms and Raspberry pi controllers. Robots moving in chain-formation
take action using motion rules based on information obtained through passive action
recognition. To find associations between robots, a regression model is created using
Deep Neural Network (DNN) and Long Short-Term Memory (LSTM), one of state-of-art technologies.
The input data of the regression model is divided into historical data, which
are consecutive positions of the robot, and observed data, which is information about the
observed robot. Historical data is sequence data that is analyzed through the LSTM
layer. The accuracy of the regression model designed using DNN can vary depending
on the quantity and quality of the input. In this thesis, three different input situations
are assumed for comparison. First, the amount of observed data is different, second, the
type of observed data is different, and third, the history length is different. Comparative
models are constructed for each case, and prediction accuracy is compared to analyze
the effect of input data on the regression model. This exploration validates that these
methods from deep learning can reduce the communication demands in coordinated
motion of multi-robot systems
can refer to the location of team robots based on information through passive action
recognition without explicit communication, various advantages (e.g. improving security
for military purposes) can be obtained. Specifically, when team robots follow
the same motion rule based on information about adjacent robots, associations can
be found between robot actions. If the association can be analyzed, this can be a clue
to the remote robot. Using these clues, it is possible to infer remote robots which are
outside of the sensor range.
In this paper, a multi-robot system is constructed using a combination of Thymio
II robotic platforms and Raspberry pi controllers. Robots moving in chain-formation
take action using motion rules based on information obtained through passive action
recognition. To find associations between robots, a regression model is created using
Deep Neural Network (DNN) and Long Short-Term Memory (LSTM), one of state-of-art technologies.
The input data of the regression model is divided into historical data, which
are consecutive positions of the robot, and observed data, which is information about the
observed robot. Historical data is sequence data that is analyzed through the LSTM
layer. The accuracy of the regression model designed using DNN can vary depending
on the quantity and quality of the input. In this thesis, three different input situations
are assumed for comparison. First, the amount of observed data is different, second, the
type of observed data is different, and third, the history length is different. Comparative
models are constructed for each case, and prediction accuracy is compared to analyze
the effect of input data on the regression model. This exploration validates that these
methods from deep learning can reduce the communication demands in coordinated
motion of multi-robot systems
Details
Title
- Comparison of Team Robot Localization by Input Difference for Deep Neural Network Model
Contributors
- Kang, Sehyeok (Author)
- Pavlic, Theodore P (Thesis advisor)
- Richa, Andréa W. (Committee member)
- Yang, Yezhou (Committee member)
- Arizona State University (Publisher)
Date Created
The date the item was original created (prior to any relationship with the ASU Digital Repositories.)
2020
Resource Type
Collections this item is in
Note
- Masters Thesis Computer Engineering 2020