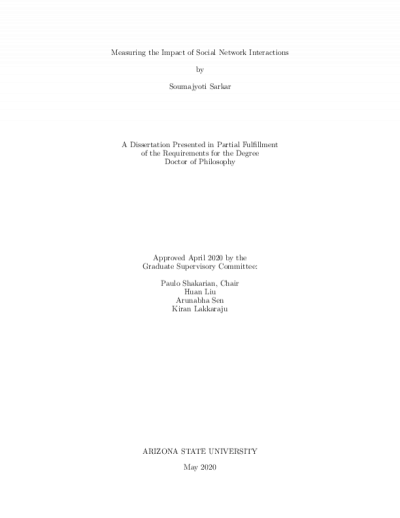
Description
Social links form the backbone of human interactions, both in an offline and online world. Such interactions harbor network diffusion or in simpler words, information spreading in a population of connected individuals. With recent increase in user engagement in social media platforms thus giving rise to networks of large scale, it has become imperative to understand the diffusion mechanisms by considering evolving instances of these network structures. Additionally, I claim that human connections fluctuate over time and attempt to study empirically grounded models of diffusion that embody these variations through evolving network structures. Patterns of interactions that are now stimulated by these fluctuating connections can be harnessed
towards predicting real world events. This dissertation attempts at analyzing
and then modeling such patterns of social network interactions. I propose how such
models could be used in advantage over traditional models of diffusion in various
predictions and simulations of real world events.
The specific three questions rooted in understanding social network interactions that have been addressed in this dissertation are: (1) can interactions captured through evolving diffusion networks indicate and predict the phase changes in a diffusion process? (2) can patterns and models of interactions in hacker forums be used in cyber-attack predictions in the real world? and (3) do varying patterns of social influence impact behavior adoption with different success ratios and could they be used to simulate rumor diffusion?
For the first question, I empirically analyze information cascades of Twitter and Flixster data and conclude that in evolving network structures characterizing diffusion, local network neighborhood surrounding a user is particularly a better indicator of the approaching phases. For the second question, I attempt to build an integrated approach utilizing unconventional signals from the "darkweb" forum discussions for predicting attacks on a target organization. The study finds that filtering out credible users and measuring network features surrounding them can be good indicators of an impending attack. For the third question, I develop an experimental framework in a controlled environment to understand how individuals respond to peer behavior in situations of sequential decision making and develop data-driven agent based models towards simulating rumor diffusion.
towards predicting real world events. This dissertation attempts at analyzing
and then modeling such patterns of social network interactions. I propose how such
models could be used in advantage over traditional models of diffusion in various
predictions and simulations of real world events.
The specific three questions rooted in understanding social network interactions that have been addressed in this dissertation are: (1) can interactions captured through evolving diffusion networks indicate and predict the phase changes in a diffusion process? (2) can patterns and models of interactions in hacker forums be used in cyber-attack predictions in the real world? and (3) do varying patterns of social influence impact behavior adoption with different success ratios and could they be used to simulate rumor diffusion?
For the first question, I empirically analyze information cascades of Twitter and Flixster data and conclude that in evolving network structures characterizing diffusion, local network neighborhood surrounding a user is particularly a better indicator of the approaching phases. For the second question, I attempt to build an integrated approach utilizing unconventional signals from the "darkweb" forum discussions for predicting attacks on a target organization. The study finds that filtering out credible users and measuring network features surrounding them can be good indicators of an impending attack. For the third question, I develop an experimental framework in a controlled environment to understand how individuals respond to peer behavior in situations of sequential decision making and develop data-driven agent based models towards simulating rumor diffusion.
Details
Title
- Measuring the Impact of Social Network Interactions
Contributors
- Sarkar, Soumajyoti (Author)
- Shakarian, Paulo (Thesis advisor)
- Liu, Huan (Committee member)
- Lakkaraju, Kiran (Committee member)
- Sen, Arunabha (Committee member)
- Arizona State University (Publisher)
Date Created
The date the item was original created (prior to any relationship with the ASU Digital Repositories.)
2020
Subjects
Resource Type
Collections this item is in
Note
- Doctoral Dissertation Computer Science 2020