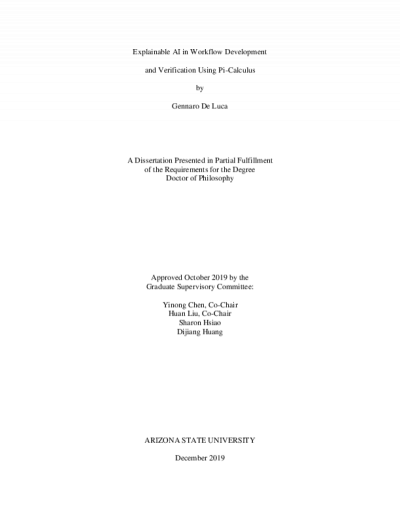
Description
Computer science education is an increasingly vital area of study with various challenges that increase the difficulty level for new students resulting in higher attrition rates. As part of an effort to resolve this issue, a new visual programming language environment was developed for this research, the Visual IoT and Robotics Programming Language Environment (VIPLE). VIPLE is based on computational thinking and flowchart, which reduces the needs of memorization of detailed syntax in text-based programming languages. VIPLE has been used at Arizona State University (ASU) in multiple years and sections of FSE100 as well as in universities worldwide. Another major issue with teaching large programming classes is the potential lack of qualified teaching assistants to grade and offer insight to a student’s programs at a level beyond output analysis.
In this dissertation, I propose a novel framework for performing semantic autograding, which analyzes student programs at a semantic level to help students learn with additional and systematic help. A general autograder is not practical for general programming languages, due to the flexibility of semantics. A practical autograder is possible in VIPLE, because of its simplified syntax and restricted options of semantics. The design of this autograder is based on the concept of theorem provers. To achieve this goal, I employ a modified version of Pi-Calculus to represent VIPLE programs and Hoare Logic to formalize program requirements. By building on the inference rules of Pi-Calculus and Hoare Logic, I am able to construct a theorem prover that can perform automated semantic analysis. Furthermore, building on this theorem prover enables me to develop a self-learning algorithm that can learn the conditions for a program’s correctness according to a given solution program.
In this dissertation, I propose a novel framework for performing semantic autograding, which analyzes student programs at a semantic level to help students learn with additional and systematic help. A general autograder is not practical for general programming languages, due to the flexibility of semantics. A practical autograder is possible in VIPLE, because of its simplified syntax and restricted options of semantics. The design of this autograder is based on the concept of theorem provers. To achieve this goal, I employ a modified version of Pi-Calculus to represent VIPLE programs and Hoare Logic to formalize program requirements. By building on the inference rules of Pi-Calculus and Hoare Logic, I am able to construct a theorem prover that can perform automated semantic analysis. Furthermore, building on this theorem prover enables me to develop a self-learning algorithm that can learn the conditions for a program’s correctness according to a given solution program.
Details
Title
- Explainable AI in Workflow Development and Verification Using Pi-Calculus
Contributors
- De Luca, Gennaro (Author)
- Chen, Yinong (Thesis advisor)
- Liu, Huan (Thesis advisor)
- Hsiao, Sharon (Committee member)
- Huang, Dijiang (Committee member)
- Arizona State University (Publisher)
Date Created
The date the item was original created (prior to any relationship with the ASU Digital Repositories.)
2020
Subjects
Resource Type
Collections this item is in
Note
- Doctoral Dissertation Computer Science 2020