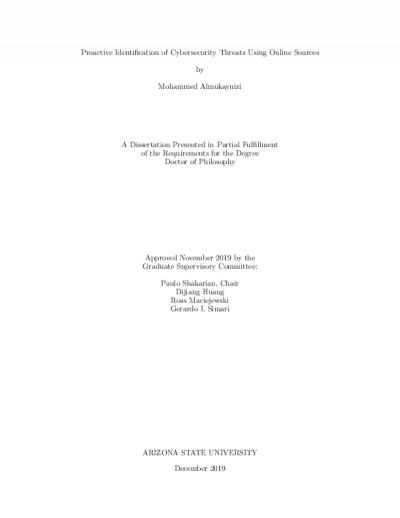
Description
Many existing applications of machine learning (ML) to cybersecurity are focused on detecting malicious activity already present in an enterprise. However, recent high-profile cyberattacks proved that certain threats could have been avoided. The speed of contemporary attacks along with the high costs of remediation incentivizes avoidance over response. Yet, avoidance implies the ability to predict - a notoriously difficult task due to high rates of false positives, difficulty in finding data that is indicative of future events, and the unexplainable results from machine learning algorithms.
In this dissertation, these challenges are addressed by presenting three artificial intelligence (AI) approaches to support prioritizing defense measures. The first two approaches leverage ML on cyberthreat intelligence data to predict if exploits are going to be used in the wild. The first work focuses on what data feeds are generated after vulnerability disclosures. The developed ML models outperform the current industry-standard method with F1 score more than doubled. Then, an approach to derive features about who generated the said data feeds is developed. The addition of these features increase recall by over 19% while maintaining precision. Finally, frequent itemset mining is combined with a variant of a probabilistic temporal logic framework to predict when attacks are likely to occur. In this approach, rules correlating malicious activity in the hacking community platforms with real-world cyberattacks are mined. They are then used in a deductive reasoning approach to generate predictions. The developed approach predicted unseen real-world attacks with an average increase in the value of F1 score by over 45%, compared to a baseline approach.
In this dissertation, these challenges are addressed by presenting three artificial intelligence (AI) approaches to support prioritizing defense measures. The first two approaches leverage ML on cyberthreat intelligence data to predict if exploits are going to be used in the wild. The first work focuses on what data feeds are generated after vulnerability disclosures. The developed ML models outperform the current industry-standard method with F1 score more than doubled. Then, an approach to derive features about who generated the said data feeds is developed. The addition of these features increase recall by over 19% while maintaining precision. Finally, frequent itemset mining is combined with a variant of a probabilistic temporal logic framework to predict when attacks are likely to occur. In this approach, rules correlating malicious activity in the hacking community platforms with real-world cyberattacks are mined. They are then used in a deductive reasoning approach to generate predictions. The developed approach predicted unseen real-world attacks with an average increase in the value of F1 score by over 45%, compared to a baseline approach.
Download count: 8
Details
Title
- Proactive Identification of Cybersecurity Threats Using Online Sources
Contributors
- Almukaynizi, Mohammed (Author)
- Shakarian, Paulo (Thesis advisor)
- Huang, Dijiang (Committee member)
- Maciejewski, Ross (Committee member)
- Simari, Gerardo I. (Committee member)
- Arizona State University (Publisher)
Date Created
The date the item was original created (prior to any relationship with the ASU Digital Repositories.)
2019
Resource Type
Collections this item is in
Note
-
Doctoral Dissertation Computer Science 2019