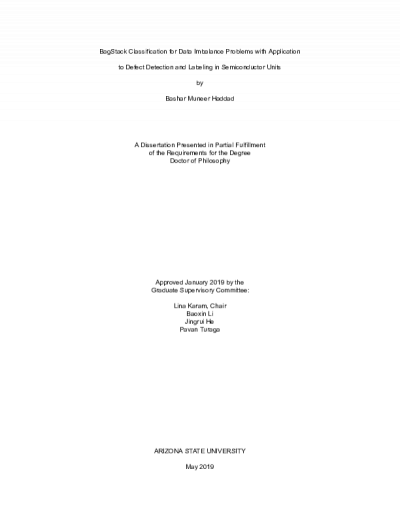
Description
Despite the fact that machine learning supports the development of computer vision applications by shortening the development cycle, finding a general learning algorithm that solves a wide range of applications is still bounded by the ”no free lunch theorem”. The search for the right algorithm to solve a specific problem is driven by the problem itself, the data availability and many other requirements.
Automated visual inspection (AVI) systems represent a major part of these challenging computer vision applications. They are gaining growing interest in the manufacturing industry to detect defective products and keep these from reaching customers. The process of defect detection and classification in semiconductor units is challenging due to different acceptable variations that the manufacturing process introduces. Other variations are also typically introduced when using optical inspection systems due to changes in lighting conditions and misalignment of the imaged units, which makes the defect detection process more challenging.
In this thesis, a BagStack classification framework is proposed, which makes use of stacking and bagging concepts to handle both variance and bias errors. The classifier is designed to handle the data imbalance and overfitting problems by adaptively transforming the
multi-class classification problem into multiple binary classification problems, applying a bagging approach to train a set of base learners for each specific problem, adaptively specifying the number of base learners assigned to each problem, adaptively specifying the number of samples to use from each class, applying a novel data-imbalance aware cross-validation technique to generate the meta-data while taking into account the data imbalance problem at the meta-data level and, finally, using a multi-response random forest regression classifier as a meta-classifier. The BagStack classifier makes use of multiple features to solve the defect classification problem. In order to detect defects, a locally adaptive statistical background modeling is proposed. The proposed BagStack classifier outperforms state-of-the-art image classification techniques on our dataset in terms of overall classification accuracy and average per-class classification accuracy. The proposed detection method achieves high performance on the considered dataset in terms of recall and precision.
Automated visual inspection (AVI) systems represent a major part of these challenging computer vision applications. They are gaining growing interest in the manufacturing industry to detect defective products and keep these from reaching customers. The process of defect detection and classification in semiconductor units is challenging due to different acceptable variations that the manufacturing process introduces. Other variations are also typically introduced when using optical inspection systems due to changes in lighting conditions and misalignment of the imaged units, which makes the defect detection process more challenging.
In this thesis, a BagStack classification framework is proposed, which makes use of stacking and bagging concepts to handle both variance and bias errors. The classifier is designed to handle the data imbalance and overfitting problems by adaptively transforming the
multi-class classification problem into multiple binary classification problems, applying a bagging approach to train a set of base learners for each specific problem, adaptively specifying the number of base learners assigned to each problem, adaptively specifying the number of samples to use from each class, applying a novel data-imbalance aware cross-validation technique to generate the meta-data while taking into account the data imbalance problem at the meta-data level and, finally, using a multi-response random forest regression classifier as a meta-classifier. The BagStack classifier makes use of multiple features to solve the defect classification problem. In order to detect defects, a locally adaptive statistical background modeling is proposed. The proposed BagStack classifier outperforms state-of-the-art image classification techniques on our dataset in terms of overall classification accuracy and average per-class classification accuracy. The proposed detection method achieves high performance on the considered dataset in terms of recall and precision.
Details
Title
- BagStack Classification for Data Imbalance Problems with Application to Defect Detection and Labeling in Semiconductor Units
Contributors
- Haddad, Bashar Muneer (Author)
- Karam, Lina (Thesis advisor)
- Li, Baoxin (Committee member)
- He, Jingrui (Committee member)
- Turaga, Pavan (Committee member)
- Arizona State University (Publisher)
Date Created
The date the item was original created (prior to any relationship with the ASU Digital Repositories.)
2019
Subjects
Resource Type
Collections this item is in
Note
- Doctoral Dissertation Computer Engineering 2019