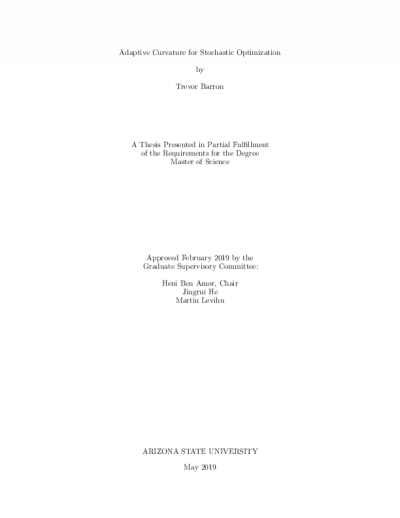
Description
This thesis presents a family of adaptive curvature methods for gradient-based stochastic optimization. In particular, a general algorithmic framework is introduced along with a practical implementation that yields an efficient, adaptive curvature gradient descent algorithm. To this end, a theoretical and practical link between curvature matrix estimation and shrinkage methods for covariance matrices is established. The use of shrinkage improves estimation accuracy of the curvature matrix when data samples are scarce. This thesis also introduce several insights that result in data- and computation-efficient update equations. Empirical results suggest that the proposed method compares favorably with existing second-order techniques based on the Fisher or Gauss-Newton and with adaptive stochastic gradient descent methods on both supervised and reinforcement learning tasks.
Details
Title
- Adaptive Curvature for Stochastic Optimization
Contributors
- Barron, Trevor (Author)
- Ben Amor, Heni (Thesis advisor)
- He, Jingrui (Committee member)
- Levihn, Martin (Committee member)
- Arizona State University (Publisher)
Date Created
The date the item was original created (prior to any relationship with the ASU Digital Repositories.)
2019
Subjects
Resource Type
Collections this item is in
Note
- thesisPartial requirement for: M.S., Arizona State University, 2019
- bibliographyIncludes bibliographical references (pages 35-39)
- Field of study: Computer science
Citation and reuse
Statement of Responsibility
by Trevor Barron