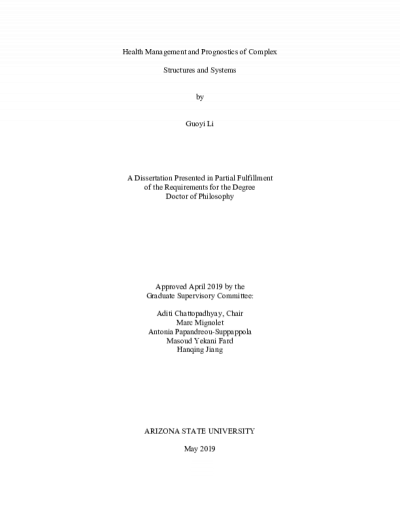
Description
This dissertation presents the development of structural health monitoring and prognostic health management methodologies for complex structures and systems in the field of mechanical engineering. To overcome various challenges historically associated with complex structures and systems such as complicated sensing mechanisms, noisy information, and large-size datasets, a hybrid monitoring framework comprising of solid mechanics concepts and data mining technologies is developed. In such a framework, the solid mechanics simulations provide additional intuitions to data mining techniques reducing the dependence of accuracy on the training set, while the data mining approaches fuse and interpret information from the targeted system enabling the capability for real-time monitoring with efficient computation.
In the case of structural health monitoring, ultrasonic guided waves are utilized for damage identification and localization in complex composite structures. Signal processing and data mining techniques are integrated into the damage localization framework, and the converted wave modes, which are induced by the thickness variation due to the presence of delamination, are used as damage indicators. This framework has been validated through experiments and has shown sufficient accuracy in locating delamination in X-COR sandwich composites without the need of baseline information. Besides the localization of internal damage, the Gaussian process machine learning technique is integrated with finite element method as an online-offline prediction model to predict crack propagation with overloads under biaxial loading conditions; such a probabilistic prognosis model, with limited number of training examples, has shown increased accuracy over state-of-the-art techniques in predicting crack retardation behaviors induced by overloads. In the case of system level management, a monitoring framework built using a multivariate Gaussian model as basis is developed to evaluate the anomalous condition of commercial aircrafts. This method has been validated using commercial airline data and has shown high sensitivity to variations in aircraft dynamics and pilot operations. Moreover, this framework was also tested on simulated aircraft faults and its feasibility for real-time monitoring was demonstrated with sufficient computation efficiency.
This research is expected to serve as a practical addition to the existing literature while possessing the potential to be adopted in realistic engineering applications.
In the case of structural health monitoring, ultrasonic guided waves are utilized for damage identification and localization in complex composite structures. Signal processing and data mining techniques are integrated into the damage localization framework, and the converted wave modes, which are induced by the thickness variation due to the presence of delamination, are used as damage indicators. This framework has been validated through experiments and has shown sufficient accuracy in locating delamination in X-COR sandwich composites without the need of baseline information. Besides the localization of internal damage, the Gaussian process machine learning technique is integrated with finite element method as an online-offline prediction model to predict crack propagation with overloads under biaxial loading conditions; such a probabilistic prognosis model, with limited number of training examples, has shown increased accuracy over state-of-the-art techniques in predicting crack retardation behaviors induced by overloads. In the case of system level management, a monitoring framework built using a multivariate Gaussian model as basis is developed to evaluate the anomalous condition of commercial aircrafts. This method has been validated using commercial airline data and has shown high sensitivity to variations in aircraft dynamics and pilot operations. Moreover, this framework was also tested on simulated aircraft faults and its feasibility for real-time monitoring was demonstrated with sufficient computation efficiency.
This research is expected to serve as a practical addition to the existing literature while possessing the potential to be adopted in realistic engineering applications.
Details
Title
- Health management and prognostics of complex structures and systems
Contributors
- Li, Guoyi (Ph.D.) (Author)
- Chattopadhyay, Aditi (Thesis advisor)
- Mignolet, Marc (Committee member)
- Papandreou-Suppappola, Antonia (Committee member)
- Yekani Fard, Masoud (Committee member)
- Jiang, Hanqing (Committee member)
- Arizona State University (Publisher)
Date Created
The date the item was original created (prior to any relationship with the ASU Digital Repositories.)
2019
Subjects
Resource Type
Collections this item is in
Note
- thesisPartial requirement for: Ph.D., Arizona State University, 2019
- bibliographyIncludes bibliographical references (pages 176-195)
- Field of study: Mechanical engineering
Citation and reuse
Statement of Responsibility
by Guoyi Li