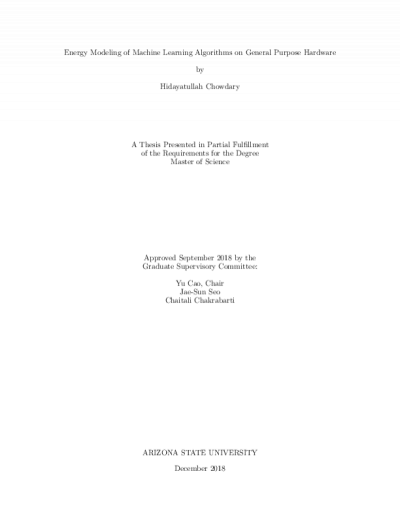
Description
Articial Neural Network(ANN) has become a for-bearer in the field of Articial Intel-
ligence. The innovations in ANN has led to ground breaking technological advances
like self-driving vehicles,medical diagnosis,speech Processing,personal assistants and
many more. These were inspired by evolution and working of our brains. Similar
to how our brain evolved using a combination of epigenetics and live stimulus,ANN
require training to learn patterns.The training usually requires a lot of computation
and memory accesses. To realize these systems in real embedded hardware many
Energy/Power/Performance issues needs to be solved. The purpose of this research
is to focus on methods to study data movement requirement for generic Neural Net-
work along with the energy associated with it and suggest some ways to improve the
design.Many methods have suggested ways to optimize using mix of computation and
data movement solutions without affecting task accuracy. But these methods lack a
computation model to calculate the energy and depend on mere back of the envelope calculation. We realized that there is a need for a generic quantitative analysis
for memory access energy which helps in better architectural exploration. We show
that the present architectural tools are either incompatible or too slow and we need
a better analytical method to estimate data movement energy. We also propose a
simplistic yet effective approach that is robust and expandable by users to support
various systems.
ligence. The innovations in ANN has led to ground breaking technological advances
like self-driving vehicles,medical diagnosis,speech Processing,personal assistants and
many more. These were inspired by evolution and working of our brains. Similar
to how our brain evolved using a combination of epigenetics and live stimulus,ANN
require training to learn patterns.The training usually requires a lot of computation
and memory accesses. To realize these systems in real embedded hardware many
Energy/Power/Performance issues needs to be solved. The purpose of this research
is to focus on methods to study data movement requirement for generic Neural Net-
work along with the energy associated with it and suggest some ways to improve the
design.Many methods have suggested ways to optimize using mix of computation and
data movement solutions without affecting task accuracy. But these methods lack a
computation model to calculate the energy and depend on mere back of the envelope calculation. We realized that there is a need for a generic quantitative analysis
for memory access energy which helps in better architectural exploration. We show
that the present architectural tools are either incompatible or too slow and we need
a better analytical method to estimate data movement energy. We also propose a
simplistic yet effective approach that is robust and expandable by users to support
various systems.
Download count: 1
Details
Title
- Energy Modeling of Machine Learning Algorithms on General Purpose Hardware
Contributors
- Chowdary, Hidayatullah (Author)
- Cao, Yu (Thesis advisor)
- Seo, JaeSun (Committee member)
- Chakrabarti, Chaitali (Committee member)
- Arizona State University (Publisher)
Date Created
The date the item was original created (prior to any relationship with the ASU Digital Repositories.)
2018
Subjects
Resource Type
Collections this item is in
Note
-
Masters Thesis Electrical Engineering 2018