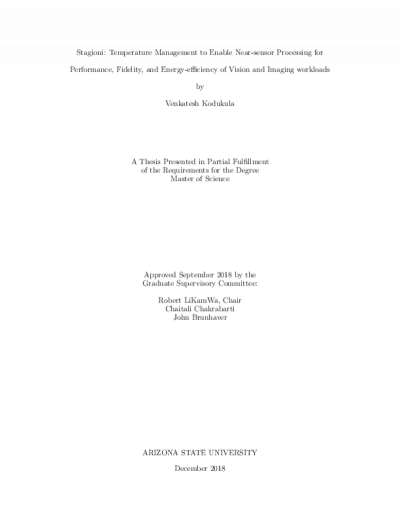
Description
Vision processing on traditional architectures is inefficient due to energy-expensive off-chip data movements. Many researchers advocate pushing processing close to the sensor to substantially reduce data movements. However, continuous near-sensor processing raises the sensor temperature, impairing the fidelity of imaging/vision tasks.
The work characterizes the thermal implications of using 3D stacked image sensors with near-sensor vision processing units. The characterization reveals that near-sensor processing reduces system power but degrades image quality. For reasonable image fidelity, the sensor temperature needs to stay below a threshold, situationally determined by application needs. Fortunately, the characterization also identifies opportunities -- unique to the needs of near-sensor processing -- to regulate temperature based on dynamic visual task requirements and rapidly increase capture quality on demand.
Based on the characterization, the work proposes and investigate two thermal management strategies -- stop-capture-go and seasonal migration -- for imaging-aware thermal management. The work present parameters that govern the policy decisions and explore the trade-offs between system power and policy overhead. The work's evaluation shows that the novel dynamic thermal management strategies can unlock the energy-efficiency potential of near-sensor processing with minimal performance impact, without compromising image fidelity.
The work characterizes the thermal implications of using 3D stacked image sensors with near-sensor vision processing units. The characterization reveals that near-sensor processing reduces system power but degrades image quality. For reasonable image fidelity, the sensor temperature needs to stay below a threshold, situationally determined by application needs. Fortunately, the characterization also identifies opportunities -- unique to the needs of near-sensor processing -- to regulate temperature based on dynamic visual task requirements and rapidly increase capture quality on demand.
Based on the characterization, the work proposes and investigate two thermal management strategies -- stop-capture-go and seasonal migration -- for imaging-aware thermal management. The work present parameters that govern the policy decisions and explore the trade-offs between system power and policy overhead. The work's evaluation shows that the novel dynamic thermal management strategies can unlock the energy-efficiency potential of near-sensor processing with minimal performance impact, without compromising image fidelity.
Download count: 1
Details
Title
- Stagioni: Temperature management to enable near-sensor processing for performance, fidelity, and energy-efficiency of vision and imaging workloads
Contributors
- Kodukula, Venkatesh (Author)
- LiKamWa, Robert (Thesis advisor)
- Chakrabarti, Chaitali (Committee member)
- Brunhaver, John (Committee member)
- Arizona State University (Publisher)
Date Created
The date the item was original created (prior to any relationship with the ASU Digital Repositories.)
2019
Subjects
Resource Type
Collections this item is in
Note
-
Masters Thesis Computer Engineering 2019