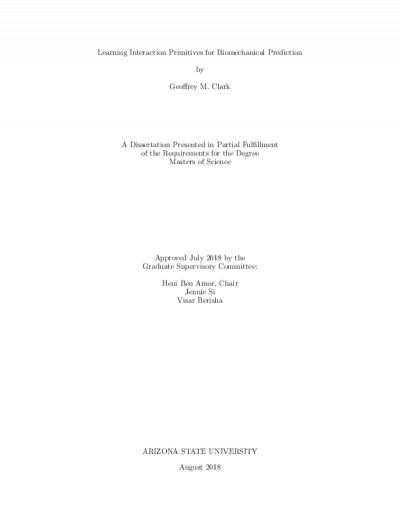
Description
This dissertation is focused on developing an algorithm to provide current state estimation and future state predictions for biomechanical human walking features. The goal is to develop a system which is capable of evaluating the current action a subject is taking while walking and then use this to predict the future states of biomechanical features.
This work focuses on the exploration and analysis of Interaction Primitives (Amor er al, 2014) and their relevance to biomechanical prediction for human walking. Built on the framework of Probabilistic Movement Primitives, Interaction Primitives utilize an EKF SLAM algorithm to localize and map a distribution over the weights of a set of basis functions. The prediction properties of Bayesian Interaction Primitives were utilized to predict real-time foot forces from a 9 degrees of freedom IMUs mounted to a subjects tibias. This method shows that real-time human biomechanical features can be predicted and have a promising link to real-time controls applications.
This work focuses on the exploration and analysis of Interaction Primitives (Amor er al, 2014) and their relevance to biomechanical prediction for human walking. Built on the framework of Probabilistic Movement Primitives, Interaction Primitives utilize an EKF SLAM algorithm to localize and map a distribution over the weights of a set of basis functions. The prediction properties of Bayesian Interaction Primitives were utilized to predict real-time foot forces from a 9 degrees of freedom IMUs mounted to a subjects tibias. This method shows that real-time human biomechanical features can be predicted and have a promising link to real-time controls applications.
Details
Title
- Learning Interaction Primitives for Biomechanical Prediction
Contributors
- Clark, Geoffrey Mitchell (Author)
- Ben Amor, Heni (Thesis advisor)
- Si, Jennie (Committee member)
- Berisha, Visar (Committee member)
- Arizona State University (Publisher)
Date Created
The date the item was original created (prior to any relationship with the ASU Digital Repositories.)
2018
Subjects
Resource Type
Collections this item is in
Note
- Masters Thesis Electrical Engineering 2018