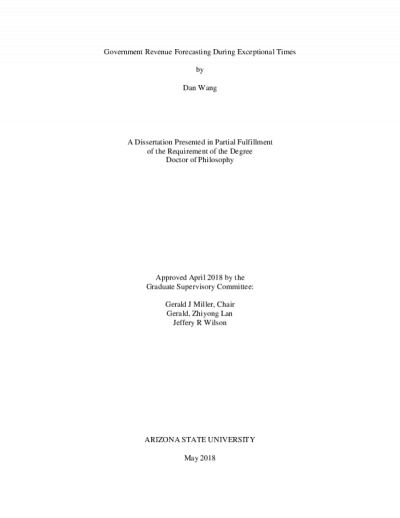
Description
Government revenue forecasting errors have become larger, especially in exceptional times such as the periods surrounding economic recessions. Inaccurate revenue estimates stem from unanticipated revenue increases or decreases from a previous trend. Unfortunately, current forecasting methods relying primarily on trend analysis do not incorporate these kinds of sudden changes easily. When revenue punctuations occur, the revenue forecasting errors increase.
To reduce forecasting errors caused by revenue punctuations in government revenue collections, I argued that analysts must not dismiss outliers as extraneous or useless phenomena. My research revealed an approach to incorporate outliers or punctuations into revenue forecasting. First, this research studied the criterion for judging the appearance of revenue punctuations using state governments’ quarterly collections of the five largest taxes from 1977 to 2016. Second, the research explored the patterns of these revenue punctuations, specifically the relationship between the changes in dollar amount and the amount of time from one revenue punctuation to another.
Inspired by the few statistical techniques for identifying outliers, this research applied the studentized residuals method to detect the revenue punctuations. The result revealed that all five tax categories for each state have revenue punctuations, except Motor Fuels Tax in the state of Tennessee.
Furthermore, this research disclosed that while not all the states and all the tax categories have statistically significant relationships between the depth and length of revenue punctuations, some states still have valid relationships. For the states that have statistically significant relationships, a forecaster, knowing depth, could calculate length and vice versa. Thus, the forecasting errors caused by revenue punctuations could be reduced when the protocols my research identified are used.
To reduce forecasting errors caused by revenue punctuations in government revenue collections, I argued that analysts must not dismiss outliers as extraneous or useless phenomena. My research revealed an approach to incorporate outliers or punctuations into revenue forecasting. First, this research studied the criterion for judging the appearance of revenue punctuations using state governments’ quarterly collections of the five largest taxes from 1977 to 2016. Second, the research explored the patterns of these revenue punctuations, specifically the relationship between the changes in dollar amount and the amount of time from one revenue punctuation to another.
Inspired by the few statistical techniques for identifying outliers, this research applied the studentized residuals method to detect the revenue punctuations. The result revealed that all five tax categories for each state have revenue punctuations, except Motor Fuels Tax in the state of Tennessee.
Furthermore, this research disclosed that while not all the states and all the tax categories have statistically significant relationships between the depth and length of revenue punctuations, some states still have valid relationships. For the states that have statistically significant relationships, a forecaster, knowing depth, could calculate length and vice versa. Thus, the forecasting errors caused by revenue punctuations could be reduced when the protocols my research identified are used.
Download count: 3
Details
Title
- Government revenue forecasting during exceptional times
Contributors
- Wang, Dan, Ph.D (Author)
- Miller, Gerald J (Thesis advisor)
- Lan, Gerald, Zhiyong (Committee member)
- Wilson, Jeffery R (Committee member)
- Arizona State University (Publisher)
Date Created
The date the item was original created (prior to any relationship with the ASU Digital Repositories.)
2018
Resource Type
Collections this item is in
Note
-
thesisPartial requirement for: Ph.D., Arizona State University, 2018
-
bibliographyIncludes bibliographical references (pages 98-101)
-
Field of study: Public Administration and Policy
Citation and reuse
Statement of Responsibility
by Dan Wang