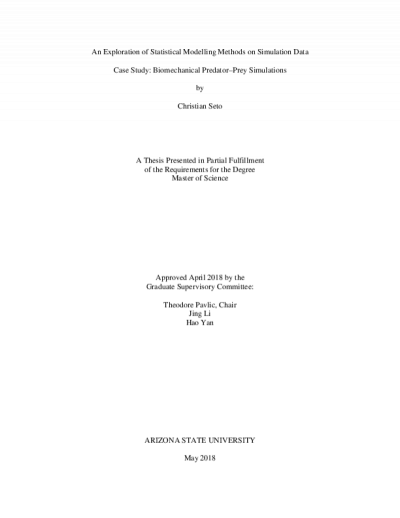
Description
Modern, advanced statistical tools from data mining and machine learning have become commonplace in molecular biology in large part because of the “big data” demands of various kinds of “-omics” (e.g., genomics, transcriptomics, metabolomics, etc.). However, in other fields of biology where empirical data sets are conventionally smaller, more traditional statistical methods of inference are still very effective and widely used. Nevertheless, with the decrease in cost of high-performance computing, these fields are starting to employ simulation models to generate insights into questions that have been elusive in the laboratory and field. Although these computational models allow for exquisite control over large numbers of parameters, they also generate data at a qualitatively different scale than most experts in these fields are accustomed to. Thus, more sophisticated methods from big-data statistics have an opportunity to better facilitate the often-forgotten area of bioinformatics that might be called “in-silicomics”.
As a case study, this thesis develops methods for the analysis of large amounts of data generated from a simulated ecosystem designed to understand how mammalian biomechanics interact with environmental complexity to modulate the outcomes of predator–prey interactions. These simulations investigate how other biomechanical parameters relating to the agility of animals in predator–prey pairs are better predictors of pursuit outcomes. Traditional modelling techniques such as forward, backward, and stepwise variable selection are initially used to study these data, but the number of parameters and potentially relevant interaction effects render these methods impractical. Consequently, new modelling techniques such as LASSO regularization are used and compared to the traditional techniques in terms of accuracy and computational complexity. Finally, the splitting rules and instances in the leaves of classification trees provide the basis for future simulation with an economical number of additional runs. In general, this thesis shows the increased utility of these sophisticated statistical techniques with simulated ecological data compared to the approaches traditionally used in these fields. These techniques combined with methods from industrial Design of Experiments will help ecologists extract novel insights from simulations that combine habitat complexity, population structure, and biomechanics.
As a case study, this thesis develops methods for the analysis of large amounts of data generated from a simulated ecosystem designed to understand how mammalian biomechanics interact with environmental complexity to modulate the outcomes of predator–prey interactions. These simulations investigate how other biomechanical parameters relating to the agility of animals in predator–prey pairs are better predictors of pursuit outcomes. Traditional modelling techniques such as forward, backward, and stepwise variable selection are initially used to study these data, but the number of parameters and potentially relevant interaction effects render these methods impractical. Consequently, new modelling techniques such as LASSO regularization are used and compared to the traditional techniques in terms of accuracy and computational complexity. Finally, the splitting rules and instances in the leaves of classification trees provide the basis for future simulation with an economical number of additional runs. In general, this thesis shows the increased utility of these sophisticated statistical techniques with simulated ecological data compared to the approaches traditionally used in these fields. These techniques combined with methods from industrial Design of Experiments will help ecologists extract novel insights from simulations that combine habitat complexity, population structure, and biomechanics.
Details
Title
- An exploration of statistical modelling methods on simulation data case study: biomechanical predator-prey simulations
Contributors
- Seto, Christian (Author)
- Pavlic, Theodore (Thesis advisor)
- Li, Jing (Committee member)
- Yan, Hao (Committee member)
- Arizona State University (Publisher)
Date Created
The date the item was original created (prior to any relationship with the ASU Digital Repositories.)
2018
Subjects
Resource Type
Collections this item is in
Note
- thesisPartial requirement for: M.S., Arizona State University, 2018
- bibliographyIncludes bibliographical references (pages 23-24)
- Field of study: Industrial engineering
Citation and reuse
Statement of Responsibility
by Christian Seto