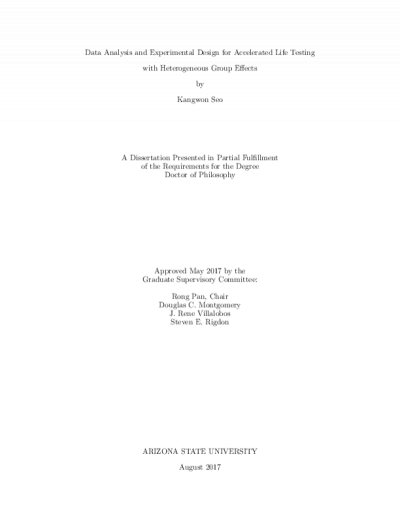
Description
In accelerated life tests (ALTs), complete randomization is hardly achievable because of economic and engineering constraints. Typical experimental protocols such as subsampling or random blocks in ALTs result in a grouped structure, which leads to correlated lifetime observations. In this dissertation, generalized linear mixed model (GLMM) approach is proposed to analyze ALT data and find the optimal ALT design with the consideration of heterogeneous group effects.
Two types of ALTs are demonstrated for data analysis. First, constant-stress ALT (CSALT) data with Weibull failure time distribution is modeled by GLMM. The marginal likelihood of observations is approximated by the quadrature rule; and the maximum likelihood (ML) estimation method is applied in iterative fashion to estimate unknown parameters including the variance component of random effect. Secondly, step-stress ALT (SSALT) data with random group effects is analyzed in similar manner but with an assumption of exponentially distributed failure time in each stress step. Two parameter estimation methods, from the frequentist’s and Bayesian points of view, are applied; and they are compared with other traditional models through simulation study and real example of the heterogeneous SSALT data. The proposed random effect model shows superiority in terms of reducing bias and variance in the estimation of life-stress relationship.
The GLMM approach is particularly useful for the optimal experimental design of ALT while taking the random group effects into account. In specific, planning ALTs under nested design structure with random test chamber effects are studied. A greedy two-phased approach shows that different test chamber assignments to stress conditions substantially impact on the estimation of unknown parameters. Then, the D-optimal test plan with two test chambers is constructed by applying the quasi-likelihood approach. Lastly, the optimal ALT planning is expanded for the case of multiple sources of random effects so that the crossed design structure is also considered, along with the nested structure.
Two types of ALTs are demonstrated for data analysis. First, constant-stress ALT (CSALT) data with Weibull failure time distribution is modeled by GLMM. The marginal likelihood of observations is approximated by the quadrature rule; and the maximum likelihood (ML) estimation method is applied in iterative fashion to estimate unknown parameters including the variance component of random effect. Secondly, step-stress ALT (SSALT) data with random group effects is analyzed in similar manner but with an assumption of exponentially distributed failure time in each stress step. Two parameter estimation methods, from the frequentist’s and Bayesian points of view, are applied; and they are compared with other traditional models through simulation study and real example of the heterogeneous SSALT data. The proposed random effect model shows superiority in terms of reducing bias and variance in the estimation of life-stress relationship.
The GLMM approach is particularly useful for the optimal experimental design of ALT while taking the random group effects into account. In specific, planning ALTs under nested design structure with random test chamber effects are studied. A greedy two-phased approach shows that different test chamber assignments to stress conditions substantially impact on the estimation of unknown parameters. Then, the D-optimal test plan with two test chambers is constructed by applying the quasi-likelihood approach. Lastly, the optimal ALT planning is expanded for the case of multiple sources of random effects so that the crossed design structure is also considered, along with the nested structure.
Download count: 2
Details
Title
- Data Analysis and Experimental Design for Accelerated Life Testing with Heterogeneous Group Effects
Contributors
- Seo, Kangwon (Author)
- Pan, Rong (Thesis advisor)
- Montgomery, Douglas C. (Committee member)
- Villalobos, J. Rene (Committee member)
- Rigdon, Steven E (Committee member)
- Arizona State University (Publisher)
Date Created
The date the item was original created (prior to any relationship with the ASU Digital Repositories.)
2017
Resource Type
Collections this item is in
Note
-
Doctoral Dissertation Industrial Engineering 2017