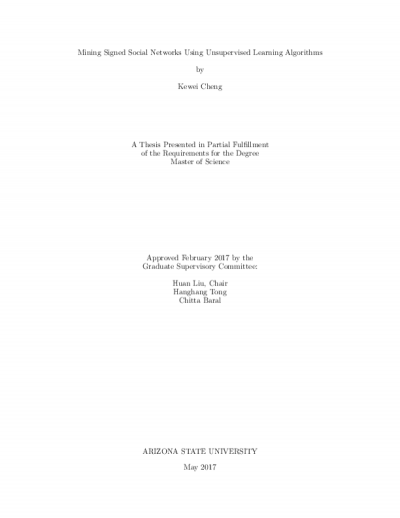
Description
Due to vast resources brought by social media services, social data mining has
received increasing attention in recent years. The availability of sheer amounts of
user-generated data presents data scientists both opportunities and challenges. Opportunities are presented with additional data sources. The abundant link information
in social networks could provide another rich source in deriving implicit information
for social data mining. However, the vast majority of existing studies overwhelmingly
focus on positive links between users while negative links are also prevailing in real-
world social networks such as distrust relations in Epinions and foe links in Slashdot.
Though recent studies show that negative links have some added value over positive
links, it is dicult to directly employ them because of its distinct characteristics from
positive interactions. Another challenge is that label information is rather limited
in social media as the labeling process requires human attention and may be very
expensive. Hence, alternative criteria are needed to guide the learning process for
many tasks such as feature selection and sentiment analysis.
To address above-mentioned issues, I study two novel problems for signed social
networks mining, (1) unsupervised feature selection in signed social networks; and
(2) unsupervised sentiment analysis with signed social networks. To tackle the first problem, I propose a novel unsupervised feature selection framework SignedFS. In
particular, I model positive and negative links simultaneously for user preference
learning, and then embed the user preference learning into feature selection. To study the second problem, I incorporate explicit sentiment signals in textual terms and
implicit sentiment signals from signed social networks into a coherent model Signed-
Senti. Empirical experiments on real-world datasets corroborate the effectiveness of
these two frameworks on the tasks of feature selection and sentiment analysis.
received increasing attention in recent years. The availability of sheer amounts of
user-generated data presents data scientists both opportunities and challenges. Opportunities are presented with additional data sources. The abundant link information
in social networks could provide another rich source in deriving implicit information
for social data mining. However, the vast majority of existing studies overwhelmingly
focus on positive links between users while negative links are also prevailing in real-
world social networks such as distrust relations in Epinions and foe links in Slashdot.
Though recent studies show that negative links have some added value over positive
links, it is dicult to directly employ them because of its distinct characteristics from
positive interactions. Another challenge is that label information is rather limited
in social media as the labeling process requires human attention and may be very
expensive. Hence, alternative criteria are needed to guide the learning process for
many tasks such as feature selection and sentiment analysis.
To address above-mentioned issues, I study two novel problems for signed social
networks mining, (1) unsupervised feature selection in signed social networks; and
(2) unsupervised sentiment analysis with signed social networks. To tackle the first problem, I propose a novel unsupervised feature selection framework SignedFS. In
particular, I model positive and negative links simultaneously for user preference
learning, and then embed the user preference learning into feature selection. To study the second problem, I incorporate explicit sentiment signals in textual terms and
implicit sentiment signals from signed social networks into a coherent model Signed-
Senti. Empirical experiments on real-world datasets corroborate the effectiveness of
these two frameworks on the tasks of feature selection and sentiment analysis.
Details
Title
- Mining signed social networks using unsupervised learning algorithms
Contributors
- Cheng, Kewei (Author)
- Liu, Huan (Thesis advisor)
- Tong, Hanghang (Committee member)
- Baral, Chitta (Committee member)
- Arizona State University (Publisher)
Date Created
The date the item was original created (prior to any relationship with the ASU Digital Repositories.)
2017
Subjects
Resource Type
Collections this item is in
Note
- thesisPartial requirement for: M.S., Arizona State University, 2017
- bibliographyIncludes bibliographical references (pages 48-51)
- Field of study: Computer science
Citation and reuse
Statement of Responsibility
by Kewei Cheng