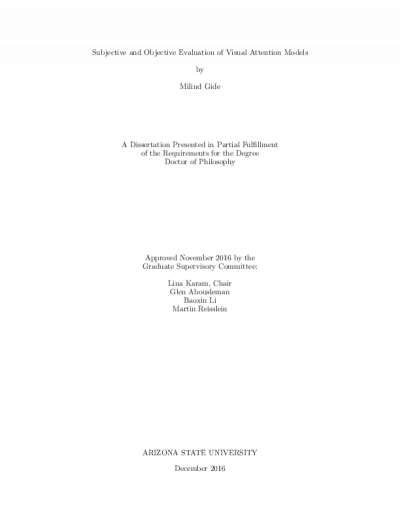
Description
Visual attention (VA) is the study of mechanisms that allow the human visual system (HVS) to selectively process relevant visual information. This work focuses on the subjective and objective evaluation of computational VA models for the distortion-free case as well as in the presence of image distortions.
Existing VA models are traditionally evaluated by using VA metrics that quantify the match between predicted saliency and fixation data obtained from eye-tracking experiments on human observers. Though there is a considerable number of objective VA metrics, there exists no study that validates that these metrics are adequate for the evaluation of VA models. This work constructs a VA Quality (VAQ) Database by subjectively assessing the prediction performance of VA models on distortion-free images. Additionally, shortcomings in existing metrics are discussed through illustrative examples and a new metric that uses local weights based on fixation density and that overcomes these flaws, is proposed. The proposed VA metric outperforms all other popular existing metrics in terms of the correlation with subjective ratings.
In practice, the image quality is affected by a host of factors at several stages of the image processing pipeline such as acquisition, compression, and transmission. However, none of the existing studies have discussed the subjective and objective evaluation of visual saliency models in the presence of distortion. In this work, a Distortion-based Visual Attention Quality (DVAQ) subjective database is constructed to evaluate the quality of VA maps for images in the presence of distortions. For creating this database, saliency maps obtained from images subjected to various types of distortions, including blur, noise and compression, and varying levels of distortion severity are rated by human observers in terms of their visual resemblance to corresponding ground-truth fixation density maps. The performance of traditionally used as well as recently proposed VA metrics are evaluated by correlating their scores with the human subjective ratings. In addition, an objective evaluation of 20 state-of-the-art VA models is performed using the top-performing VA metrics together with a study of how the VA models’ prediction performance changes with different types and levels of distortions.
Existing VA models are traditionally evaluated by using VA metrics that quantify the match between predicted saliency and fixation data obtained from eye-tracking experiments on human observers. Though there is a considerable number of objective VA metrics, there exists no study that validates that these metrics are adequate for the evaluation of VA models. This work constructs a VA Quality (VAQ) Database by subjectively assessing the prediction performance of VA models on distortion-free images. Additionally, shortcomings in existing metrics are discussed through illustrative examples and a new metric that uses local weights based on fixation density and that overcomes these flaws, is proposed. The proposed VA metric outperforms all other popular existing metrics in terms of the correlation with subjective ratings.
In practice, the image quality is affected by a host of factors at several stages of the image processing pipeline such as acquisition, compression, and transmission. However, none of the existing studies have discussed the subjective and objective evaluation of visual saliency models in the presence of distortion. In this work, a Distortion-based Visual Attention Quality (DVAQ) subjective database is constructed to evaluate the quality of VA maps for images in the presence of distortions. For creating this database, saliency maps obtained from images subjected to various types of distortions, including blur, noise and compression, and varying levels of distortion severity are rated by human observers in terms of their visual resemblance to corresponding ground-truth fixation density maps. The performance of traditionally used as well as recently proposed VA metrics are evaluated by correlating their scores with the human subjective ratings. In addition, an objective evaluation of 20 state-of-the-art VA models is performed using the top-performing VA metrics together with a study of how the VA models’ prediction performance changes with different types and levels of distortions.
Details
Title
- Subjective and objective evaluation of visual attention models
Contributors
- Gide, Milind Subhash (Author)
- Karam, Lina J (Thesis advisor)
- Abousleman, Glen (Committee member)
- Li, Baoxin (Committee member)
- Reisslein, Martin (Committee member)
- Arizona State University (Publisher)
Date Created
The date the item was original created (prior to any relationship with the ASU Digital Repositories.)
2016
Subjects
Resource Type
Collections this item is in
Note
-
thesisPartial requirement for: Ph.D., Arizona State University, 2016
-
bibliographyIncludes bibliographical references (pages 77-81)
-
Field of study: Electrical engineering
Citation and reuse
Statement of Responsibility
by Milind Subhash Gide