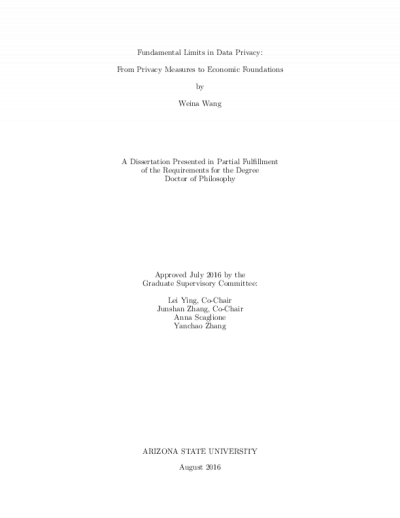
Description
Data privacy is emerging as one of the most serious concerns of big data analytics, particularly with the growing use of personal data and the ever-improving capability of data analysis. This dissertation first investigates the relation between different privacy notions, and then puts the main focus on developing economic foundations for a market model of trading private data.
The first part characterizes differential privacy, identifiability and mutual-information privacy by their privacy--distortion functions, which is the optimal achievable privacy level as a function of the maximum allowable distortion. The results show that these notions are fundamentally related and exhibit certain consistency: (1) The gap between the privacy--distortion functions of identifiability and differential privacy is upper bounded by a constant determined by the prior. (2) Identifiability and mutual-information privacy share the same optimal mechanism. (3) The mutual-information optimal mechanism satisfies differential privacy with a level at most a constant away from the optimal level.
The second part studies a market model of trading private data, where a data collector purchases private data from strategic data subjects (individuals) through an incentive mechanism. The value of epsilon units of privacy is measured by the minimum payment such that an individual's equilibrium strategy is to report data in an epsilon-differentially private manner. For the setting with binary private data that represents individuals' knowledge about a common underlying state, asymptotically tight lower and upper bounds on the value of privacy are established as the number of individuals becomes large, and the payment--accuracy tradeoff for learning the state is obtained. The lower bound assures the impossibility of using lower payment to buy epsilon units of privacy, and the upper bound is given by a designed reward mechanism. When the individuals' valuations of privacy are unknown to the data collector, mechanisms with possible negative payments (aiming to penalize individuals with "unacceptably" high privacy valuations) are designed to fulfill the accuracy goal and drive the total payment to zero. For the setting with binary private data following a general joint probability distribution with some symmetry, asymptotically optimal mechanisms are designed in the high data quality regime.
The first part characterizes differential privacy, identifiability and mutual-information privacy by their privacy--distortion functions, which is the optimal achievable privacy level as a function of the maximum allowable distortion. The results show that these notions are fundamentally related and exhibit certain consistency: (1) The gap between the privacy--distortion functions of identifiability and differential privacy is upper bounded by a constant determined by the prior. (2) Identifiability and mutual-information privacy share the same optimal mechanism. (3) The mutual-information optimal mechanism satisfies differential privacy with a level at most a constant away from the optimal level.
The second part studies a market model of trading private data, where a data collector purchases private data from strategic data subjects (individuals) through an incentive mechanism. The value of epsilon units of privacy is measured by the minimum payment such that an individual's equilibrium strategy is to report data in an epsilon-differentially private manner. For the setting with binary private data that represents individuals' knowledge about a common underlying state, asymptotically tight lower and upper bounds on the value of privacy are established as the number of individuals becomes large, and the payment--accuracy tradeoff for learning the state is obtained. The lower bound assures the impossibility of using lower payment to buy epsilon units of privacy, and the upper bound is given by a designed reward mechanism. When the individuals' valuations of privacy are unknown to the data collector, mechanisms with possible negative payments (aiming to penalize individuals with "unacceptably" high privacy valuations) are designed to fulfill the accuracy goal and drive the total payment to zero. For the setting with binary private data following a general joint probability distribution with some symmetry, asymptotically optimal mechanisms are designed in the high data quality regime.
Details
Title
- Fundamental limits in data privacy: from privacy measures to economic foundations
Contributors
- Wang, Weina (Author)
- Ying, Lei (Thesis advisor)
- Zhang, Junshan (Thesis advisor)
- Scaglione, Anna (Committee member)
- Zhang, Yanchao (Committee member)
- Arizona State University (Publisher)
Date Created
The date the item was original created (prior to any relationship with the ASU Digital Repositories.)
2016
Subjects
Resource Type
Collections this item is in
Note
- thesisPartial requirement for: Ph.D., Arizona State University, 2016
- bibliographyIncludes bibliographical references (pages 106-111)
- Field of study: Electrical engineering
Citation and reuse
Statement of Responsibility
by Weina Wang