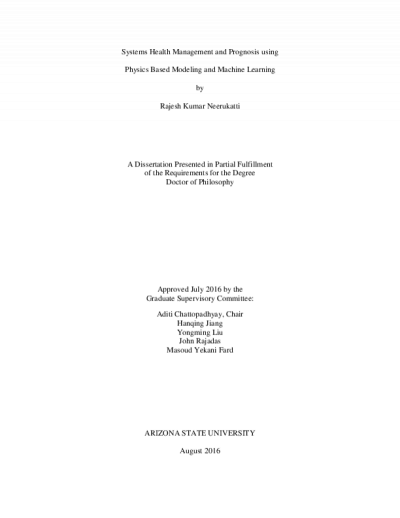
Description
There is a concerted effort in developing robust systems health monitoring/management (SHM) technology as a means to reduce the life cycle costs, improve availability, extend life and minimize downtime of various platforms including aerospace and civil infrastructure. The implementation of a robust SHM system requires a collaborative effort in a variety of areas such as sensor development, damage detection and localization, physics based models, and prognosis models for residual useful life (RUL) estimation. Damage localization and prediction is further complicated by geometric, material, loading, and environmental variabilities. Therefore, it is essential to develop robust SHM methodologies by taking into account such uncertainties. In this research, damage localization and RUL estimation of two different physical systems are addressed: (i) fatigue crack propagation in metallic materials under complex multiaxial loading and (ii) temporal scour prediction near bridge piers. With little modifications, the methodologies developed can be applied to other systems.
Current practice in fatigue life prediction is based on either physics based modeling or data-driven methods, and is limited to predicting RUL for simple geometries under uniaxial loading conditions. In this research, crack initiation and propagation behavior under uniaxial and complex biaxial fatigue loading is addressed. The crack propagation behavior is studied by performing extensive material characterization and fatigue testing under in-plane biaxial loading, both in-phase and out-of-phase, with different biaxiality ratios. A hybrid prognosis model, which combines machine learning with physics based modeling, is developed to account for the uncertainties in crack propagation and fatigue life prediction due to variabilities in material microstructural characteristics, crack localization information and environmental changes. The methodology iteratively combines localization information with hybrid prognosis models using sequential Bayesian techniques. The results show significant improvements in the localization and prediction accuracy under varying temperature.
For civil infrastructure, especially bridges, pier scour is a major failure mechanism. Currently available techniques are developed from a design perspective and provide highly conservative scour estimates. In this research, a fully probabilistic scour prediction methodology is developed using machine learning to accurately predict scour in real-time under varying flow conditions.
Current practice in fatigue life prediction is based on either physics based modeling or data-driven methods, and is limited to predicting RUL for simple geometries under uniaxial loading conditions. In this research, crack initiation and propagation behavior under uniaxial and complex biaxial fatigue loading is addressed. The crack propagation behavior is studied by performing extensive material characterization and fatigue testing under in-plane biaxial loading, both in-phase and out-of-phase, with different biaxiality ratios. A hybrid prognosis model, which combines machine learning with physics based modeling, is developed to account for the uncertainties in crack propagation and fatigue life prediction due to variabilities in material microstructural characteristics, crack localization information and environmental changes. The methodology iteratively combines localization information with hybrid prognosis models using sequential Bayesian techniques. The results show significant improvements in the localization and prediction accuracy under varying temperature.
For civil infrastructure, especially bridges, pier scour is a major failure mechanism. Currently available techniques are developed from a design perspective and provide highly conservative scour estimates. In this research, a fully probabilistic scour prediction methodology is developed using machine learning to accurately predict scour in real-time under varying flow conditions.
Download count: 5
Details
Title
- Systems health management and prognosis using physics based modeling and machine learning
Contributors
- Neerukatti, Rajesh Kumar (Author)
- Chattopadhyay, Aditi (Thesis advisor)
- Jiang, Hanqing (Committee member)
- Liu, Yongming (Committee member)
- Rajadas, John (Committee member)
- Yekani Fard, Masoud (Committee member)
- Arizona State University (Publisher)
Date Created
The date the item was original created (prior to any relationship with the ASU Digital Repositories.)
2016
Subjects
Resource Type
Collections this item is in
Note
-
thesisPartial requirement for: Ph.D., Arizona State University, 2016
-
bibliographyIncludes bibliographical references (pages 180-192)
-
Field of study: Mechanical engineering
Citation and reuse
Statement of Responsibility
by Rajesh Kumar Neerukatti