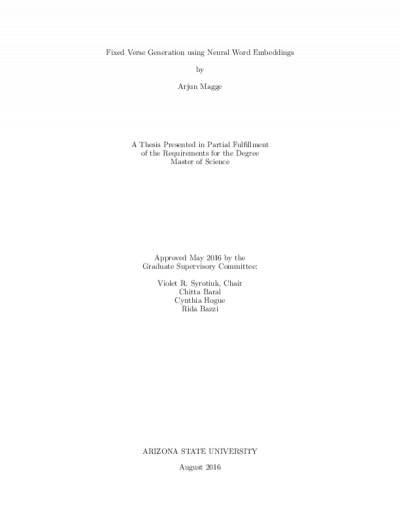
Description
For the past three decades, the design of an effective strategy for generating poetry that matches that of a human’s creative capabilities and complexities has been an elusive goal in artificial intelligence (AI) and natural language generation (NLG) research, and among linguistic creativity researchers in particular. This thesis presents a novel approach to fixed verse poetry generation using neural word embeddings. During the course of generation, a two layered poetry classifier is developed. The first layer uses a lexicon based method to classify poems into types based on form and structure, and the second layer uses a supervised classification method to classify poems into subtypes based on content with an accuracy of 92%. The system then uses a two-layer neural network to generate poetry based on word similarities and word movements in a 50-dimensional vector space.
The verses generated by the system are evaluated using rhyme, rhythm, syllable counts and stress patterns. These computational features of language are considered for generating haikus, limericks and iambic pentameter verses. The generated poems are evaluated using a Turing test on both experts and non-experts. The user study finds that only 38% computer generated poems were correctly identified by nonexperts while 65% of the computer generated poems were correctly identified by experts. Although the system does not pass the Turing test, the results from the Turing test suggest an improvement of over 17% when compared to previous methods which use Turing tests to evaluate poetry generators.
The verses generated by the system are evaluated using rhyme, rhythm, syllable counts and stress patterns. These computational features of language are considered for generating haikus, limericks and iambic pentameter verses. The generated poems are evaluated using a Turing test on both experts and non-experts. The user study finds that only 38% computer generated poems were correctly identified by nonexperts while 65% of the computer generated poems were correctly identified by experts. Although the system does not pass the Turing test, the results from the Turing test suggest an improvement of over 17% when compared to previous methods which use Turing tests to evaluate poetry generators.
Details
Title
- Fixed verse generation using neural word embeddings
Contributors
- Magge, Arjun (Author)
- Syrotiuk, Violet R. (Thesis advisor)
- Baral, Chitta (Committee member)
- Hogue, Cynthia (Committee member)
- Bazzi, Rida (Committee member)
- Arizona State University (Publisher)
Date Created
The date the item was original created (prior to any relationship with the ASU Digital Repositories.)
2016
Subjects
Resource Type
Collections this item is in
Note
- thesisPartial requirement for: M.S., Arizona State University, 2016
- bibliographyIncludes bibliographical references (pages 60-67)
- Field of study: Computer science
Citation and reuse
Statement of Responsibility
by Arjun Magge