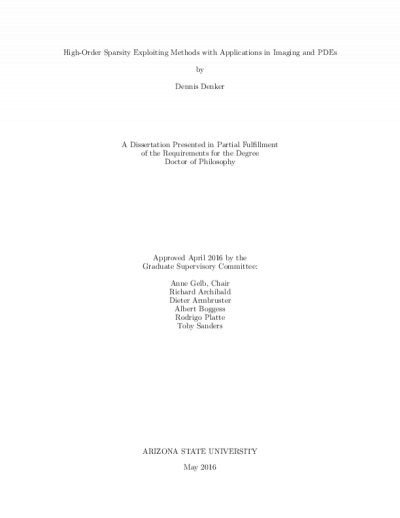
Description
High-order methods are known for their accuracy and computational performance when applied to solving partial differential equations and have widespread use
in representing images compactly. Nonetheless, high-order methods have difficulty representing functions containing discontinuities or functions having slow spectral decay in the chosen basis. Certain sensing techniques such as MRI and SAR provide data in terms of Fourier coefficients, and thus prescribe a natural high-order basis. The field of compressed sensing has introduced a set of techniques based on $\ell^1$ regularization that promote sparsity and facilitate working with functions having discontinuities. In this dissertation, high-order methods and $\ell^1$ regularization are used to address three problems: reconstructing piecewise smooth functions from sparse and and noisy Fourier data, recovering edge locations in piecewise smooth functions from sparse and noisy Fourier data, and reducing time-stepping constraints when numerically solving certain time-dependent hyperbolic partial differential equations.
in representing images compactly. Nonetheless, high-order methods have difficulty representing functions containing discontinuities or functions having slow spectral decay in the chosen basis. Certain sensing techniques such as MRI and SAR provide data in terms of Fourier coefficients, and thus prescribe a natural high-order basis. The field of compressed sensing has introduced a set of techniques based on $\ell^1$ regularization that promote sparsity and facilitate working with functions having discontinuities. In this dissertation, high-order methods and $\ell^1$ regularization are used to address three problems: reconstructing piecewise smooth functions from sparse and and noisy Fourier data, recovering edge locations in piecewise smooth functions from sparse and noisy Fourier data, and reducing time-stepping constraints when numerically solving certain time-dependent hyperbolic partial differential equations.
Download count: 4
Details
Title
- High-order sparsity exploiting methods with applications in imaging and PDEs
Contributors
- Denker, Dennis (Author)
- Gelb, Anne (Thesis advisor)
- Archibald, Richard (Committee member)
- Armbruster, Dieter (Committee member)
- Boggess, Albert (Committee member)
- Platte, Rodrigo (Committee member)
- Saders, Toby (Committee member)
- Arizona State University (Publisher)
Date Created
The date the item was original created (prior to any relationship with the ASU Digital Repositories.)
2016
Subjects
Resource Type
Collections this item is in
Note
-
thesisPartial requirement for: Ph.D., Arizona State University, 2016
-
bibliographyIncludes bibliographical references (pages 105-108)
-
Field of study: Applied mathematics
Citation and reuse
Statement of Responsibility
by Dennis Denker