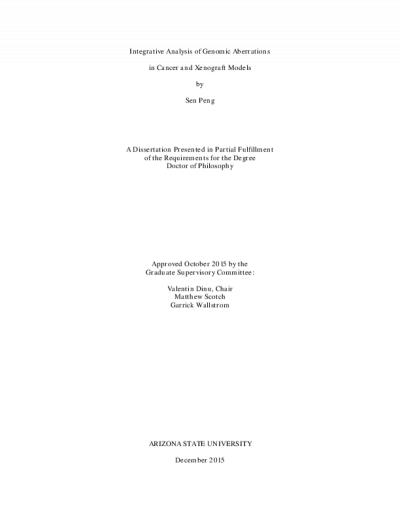
Description
No two cancers are alike. Cancer is a dynamic and heterogeneous disease, such heterogeneity arise among patients with the same cancer type, among cancer cells within the same individual’s tumor and even among cells within the same sub-clone over time. The recent application of next-generation sequencing and precision medicine techniques is the driving force to uncover the complexity of cancer and the best clinical practice. The core concept of precision medicine is to move away from crowd-based, best-for-most treatment and take individual variability into account when optimizing the prevention and treatment strategies. Next-generation sequencing is the method to sift through the entire 3 billion letters of each patient’s DNA genetic code in a massively parallel fashion.
The deluge of next-generation sequencing data nowadays has shifted the bottleneck of cancer research from multiple “-omics” data collection to integrative analysis and data interpretation. In this dissertation, I attempt to address two distinct, but dependent, challenges. The first is to design specific computational algorithms and tools that can process and extract useful information from the raw data in an efficient, robust, and reproducible manner. The second challenge is to develop high-level computational methods and data frameworks for integrating and interpreting these data. Specifically, Chapter 2 presents a tool called Snipea (SNv Integration, Prioritization, Ensemble, and Annotation) to further identify, prioritize and annotate somatic SNVs (Single Nucleotide Variant) called from multiple variant callers. Chapter 3 describes a novel alignment-based algorithm to accurately and losslessly classify sequencing reads from xenograft models. Chapter 4 describes a direct and biologically motivated framework and associated methods for identification of putative aberrations causing survival difference in GBM patients by integrating whole-genome sequencing, exome sequencing, RNA-Sequencing, methylation array and clinical data. Lastly, chapter 5 explores longitudinal and intratumor heterogeneity studies to reveal the temporal and spatial context of tumor evolution. The long-term goal is to help patients with cancer, particularly those who are in front of us today. Genome-based analysis of the patient tumor can identify genomic alterations unique to each patient’s tumor that are candidate therapeutic targets to decrease therapy resistance and improve clinical outcome.
The deluge of next-generation sequencing data nowadays has shifted the bottleneck of cancer research from multiple “-omics” data collection to integrative analysis and data interpretation. In this dissertation, I attempt to address two distinct, but dependent, challenges. The first is to design specific computational algorithms and tools that can process and extract useful information from the raw data in an efficient, robust, and reproducible manner. The second challenge is to develop high-level computational methods and data frameworks for integrating and interpreting these data. Specifically, Chapter 2 presents a tool called Snipea (SNv Integration, Prioritization, Ensemble, and Annotation) to further identify, prioritize and annotate somatic SNVs (Single Nucleotide Variant) called from multiple variant callers. Chapter 3 describes a novel alignment-based algorithm to accurately and losslessly classify sequencing reads from xenograft models. Chapter 4 describes a direct and biologically motivated framework and associated methods for identification of putative aberrations causing survival difference in GBM patients by integrating whole-genome sequencing, exome sequencing, RNA-Sequencing, methylation array and clinical data. Lastly, chapter 5 explores longitudinal and intratumor heterogeneity studies to reveal the temporal and spatial context of tumor evolution. The long-term goal is to help patients with cancer, particularly those who are in front of us today. Genome-based analysis of the patient tumor can identify genomic alterations unique to each patient’s tumor that are candidate therapeutic targets to decrease therapy resistance and improve clinical outcome.
Download count: 1
Details
Title
- Integrative analysis of genomic aberrations in cancer and xenograft Models
Contributors
- Peng, Sen (Author)
- Dinu, Valentin (Thesis advisor)
- Scotch, Matthew (Committee member)
- Wallstrom, Garrick (Committee member)
- Arizona State University (Publisher)
Date Created
The date the item was original created (prior to any relationship with the ASU Digital Repositories.)
2015
Subjects
Resource Type
Collections this item is in
Note
-
thesisPartial requirement for: Ph.D., Arizona State University, 2015
-
bibliographyIncludes bibliographical references (pages 123-132)
-
Field of study: Biomedical informatics
Citation and reuse
Statement of Responsibility
by Sen Peng