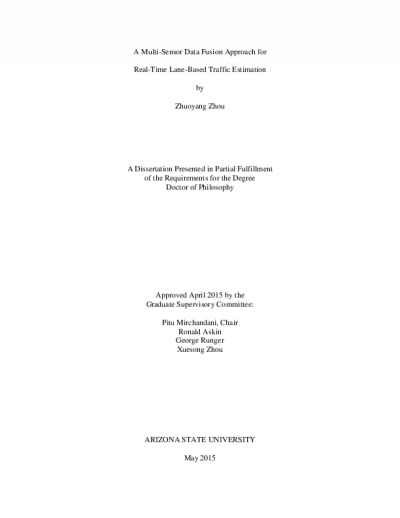
Description
Modern intelligent transportation systems (ITS) make driving more efficient, easier, and safer. Knowledge of real-time traffic conditions is a critical input for operating ITS. Real-time freeway traffic state estimation approaches have been used to quantify traffic conditions given limited amount of data collected by traffic sensors. Currently, almost all real-time estimation methods have been developed for estimating laterally aggregated traffic conditions in a roadway segment using link-based models which assume homogeneous conditions across multiple lanes. However, with new advances and applications of ITS, knowledge of lane-based traffic conditions is becoming important, where the traffic condition differences among lanes are recognized. In addition, most of the current real-time freeway traffic estimators consider only data from loop detectors. This dissertation develops a bi-level data fusion approach using heterogeneous multi-sensor measurements to estimate real-time lane-based freeway traffic conditions, which integrates a link-level model-based estimator and a lane-level data-driven estimator.
Macroscopic traffic flow models describe the evolution of aggregated traffic characteristics over time and space, which are required by model-based traffic estimation approaches. Since current first-order Lagrangian macroscopic traffic flow model has some unrealistic implicit assumptions (e.g., infinite acceleration), a second-order Lagrangian macroscopic traffic flow model has been developed by incorporating drivers’ anticipation and reaction delay. A multi-sensor extended Kalman filter (MEKF) algorithm has been developed to combine heterogeneous measurements from multiple sources. A MEKF-based traffic estimator, explicitly using the developed second-order traffic flow model and measurements from loop detectors as well as GPS trajectories for given fractions of vehicles, has been proposed which gives real-time link-level traffic estimates in the bi-level estimation system.
The lane-level estimation in the bi-level data fusion system uses the link-level estimates as priors and adopts a data-driven approach to obtain lane-based estimates, where now heterogeneous multi-sensor measurements are combined using parallel spatial-temporal filters.
Experimental analysis shows that the second-order model can more realistically reproduce real world traffic flow patterns (e.g., stop-and-go waves). The MEKF-based link-level estimator exhibits more accurate results than the estimator that uses only a single data source. Evaluation of the lane-level estimator demonstrates that the proposed new bi-level multi-sensor data fusion system can provide very good estimates of real-time lane-based traffic conditions.
Macroscopic traffic flow models describe the evolution of aggregated traffic characteristics over time and space, which are required by model-based traffic estimation approaches. Since current first-order Lagrangian macroscopic traffic flow model has some unrealistic implicit assumptions (e.g., infinite acceleration), a second-order Lagrangian macroscopic traffic flow model has been developed by incorporating drivers’ anticipation and reaction delay. A multi-sensor extended Kalman filter (MEKF) algorithm has been developed to combine heterogeneous measurements from multiple sources. A MEKF-based traffic estimator, explicitly using the developed second-order traffic flow model and measurements from loop detectors as well as GPS trajectories for given fractions of vehicles, has been proposed which gives real-time link-level traffic estimates in the bi-level estimation system.
The lane-level estimation in the bi-level data fusion system uses the link-level estimates as priors and adopts a data-driven approach to obtain lane-based estimates, where now heterogeneous multi-sensor measurements are combined using parallel spatial-temporal filters.
Experimental analysis shows that the second-order model can more realistically reproduce real world traffic flow patterns (e.g., stop-and-go waves). The MEKF-based link-level estimator exhibits more accurate results than the estimator that uses only a single data source. Evaluation of the lane-level estimator demonstrates that the proposed new bi-level multi-sensor data fusion system can provide very good estimates of real-time lane-based traffic conditions.
Details
Title
- A multi-sensor data fusion approach for real-time lane-based traffic estimation
Contributors
- Zhou, Zhuoyang (Author)
- Mirchandani, Pitu (Thesis advisor)
- Askin, Ronald (Committee member)
- Runger, George C. (Committee member)
- Zhou, Xuesong (Committee member)
- Arizona State University (Publisher)
Date Created
The date the item was original created (prior to any relationship with the ASU Digital Repositories.)
2015
Subjects
Resource Type
Collections this item is in
Note
- thesisPartial requirement for: Ph.D., Arizona State University, 2015
- bibliographyIncludes bibliographical references (pages 163-170)
- Field of study: Industrial engineering
Citation and reuse
Statement of Responsibility
by Zhuoyang Zhou