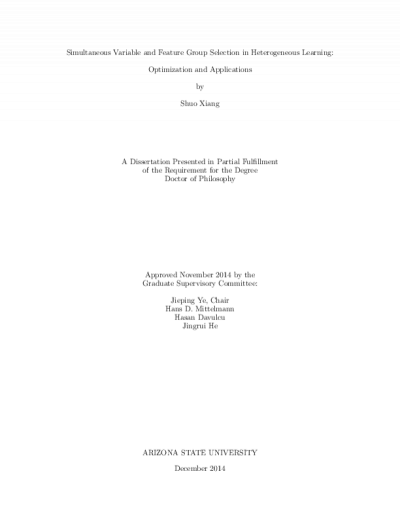
Description
Advances in data collection technologies have made it cost-effective to obtain heterogeneous data from multiple data sources. Very often, the data are of very high dimension and feature selection is preferred in order to reduce noise, save computational cost and learn interpretable models. Due to the multi-modality nature of heterogeneous data, it is interesting to design efficient machine learning models that are capable of performing variable selection and feature group (data source) selection simultaneously (a.k.a bi-level selection). In this thesis, I carry out research along this direction with a particular focus on designing efficient optimization algorithms. I start with a unified bi-level learning model that contains several existing feature selection models as special cases. Then the proposed model is further extended to tackle the block-wise missing data, one of the major challenges in the diagnosis of Alzheimer's Disease (AD). Moreover, I propose a novel interpretable sparse group feature selection model that greatly facilitates the procedure of parameter tuning and model selection. Last but not least, I show that by solving the sparse group hard thresholding problem directly, the sparse group feature selection model can be further improved in terms of both algorithmic complexity and efficiency. Promising results are demonstrated in the extensive evaluation on multiple real-world data sets.
Details
Title
- Simultaneous variable and feature group selection in heterogeneous learning: optimization and applications
Contributors
- Xiang, Shuo (Author)
- Ye, Jieping (Thesis advisor)
- Mittelmann, Hans D (Committee member)
- Davulcu, Hasan (Committee member)
- He, Jingrui (Committee member)
- Arizona State University (Publisher)
Date Created
The date the item was original created (prior to any relationship with the ASU Digital Repositories.)
2014
Subjects
Resource Type
Collections this item is in
Note
- thesisPartial requirement for: Ph.D., Arizona State University, 2014
- bibliographyIncludes bibliographical references (p. 84-90)
- Field of study: Computer science
Citation and reuse
Statement of Responsibility
by Shuo Xiang