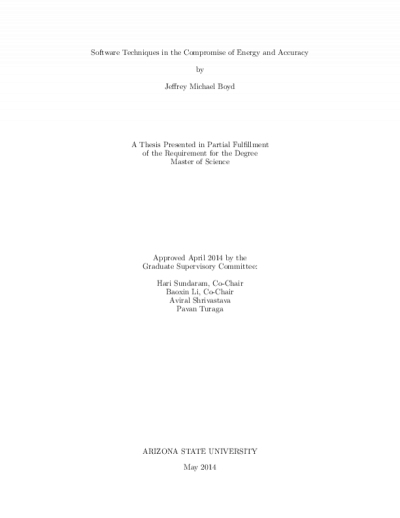
Description
Software has a great impact on the energy efficiency of any computing system--it can manage the components of a system efficiently or inefficiently. The impact of software is amplified in the context of a wearable computing system used for activity recognition. The design space this platform opens up is immense and encompasses sensors, feature calculations, activity classification algorithms, sleep schedules, and transmission protocols. Design choices in each of these areas impact energy use, overall accuracy, and usefulness of the system. This thesis explores methods software can influence the trade-off between energy consumption and system accuracy. In general the more energy a system consumes the more accurate will be. We explore how finding the transitions between human activities is able to reduce the energy consumption of such systems without reducing much accuracy. We introduce the Log-likelihood Ratio Test as a method to detect transitions, and explore how choices of sensor, feature calculations, and parameters concerning time segmentation affect the accuracy of this method. We discovered an approximate 5X increase in energy efficiency could be achieved with only a 5% decrease in accuracy. We also address how a system's sleep mode, in which the processor enters a low-power state and sensors are turned off, affects a wearable computing platform that does activity recognition. We discuss the energy trade-offs in each stage of the activity recognition process. We find that careful analysis of these parameters can result in great increases in energy efficiency if small compromises in overall accuracy can be tolerated. We call this the ``Great Compromise.'' We found a 6X increase in efficiency with a 7% decrease in accuracy. We then consider how wireless transmission of data affects the overall energy efficiency of a wearable computing platform. We find that design decisions such as feature calculations and grouping size have a great impact on the energy consumption of the system because of the amount of data that is stored and transmitted. For example, storing and transmitting vector-based features such as FFT or DCT do not compress the signal and would use more energy than storing and transmitting the raw signal. The effect of grouping size on energy consumption depends on the feature. For scalar features energy consumption is proportional in the inverse of grouping size, so it's reduced as grouping size goes up. For features that depend on the grouping size, such as FFT, energy increases with the logarithm of grouping size, so energy consumption increases slowly as grouping size increases. We find that compressing data through activity classification and transition detection significantly reduces energy consumption and that the energy consumed for the classification overhead is negligible compared to the energy savings from data compression. We provide mathematical models of energy usage and data generation, and test our ideas using a mobile computing platform, the Texas Instruments Chronos watch.
Details
Title
- Software techniques in the compromise of energy and accuracy
Contributors
- Boyd, Jeffrey Michael (Author)
- Sundaram, Hari (Thesis advisor)
- Li, Baoxin (Thesis advisor)
- Shrivastava, Aviral (Committee member)
- Turaga, Pavan (Committee member)
- Arizona State University (Publisher)
Date Created
The date the item was original created (prior to any relationship with the ASU Digital Repositories.)
2014
Subjects
Resource Type
Collections this item is in
Note
-
thesisPartial requirement for: M.S., Arizona State University, 2014
-
bibliographyIncludes bibliographical references (p. 80-82)
-
Field of study: Computer science
Citation and reuse
Statement of Responsibility
by Jeffrey Michael Boyd