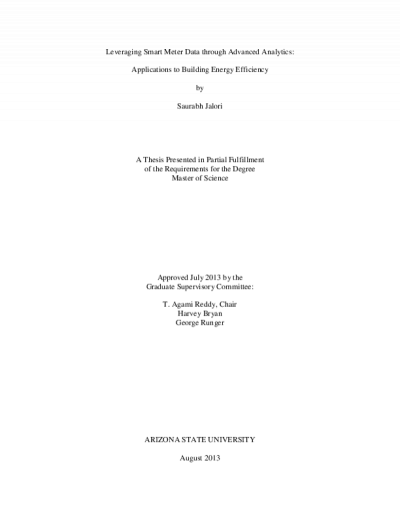
Description
The poor energy efficiency of buildings is a major barrier to alleviating the energy dilemma. Historically, monthly utility billing data was widely available and analytical methods for identifying building energy efficiency improvements, performing building Monitoring and Verification (M&V;) and continuous commissioning (CCx) were based on them. Although robust, these methods were not sensitive enough to detect a number of common causes for increased energy use. In recent years, prevalence of short-term building energy consumption data, also known as Energy Interval Data (EID), made available through the Smart Meters, along with data mining techniques presents the potential of knowledge discovery inherent in this data. This allows more sophisticated analytical tools to be developed resulting in greater sensitivities due to higher prediction accuracies; leading to deep energy savings and highly efficient building system operations. The research explores enhancements to Inverse Statistical Modeling techniques due to the availability of EID. Inverse statistical modeling is the process of identification of prediction model structure and estimates of model parameters. The methodology is based on several common statistical and data mining techniques: cluster analysis for day typing, outlier detection and removal, and generation of building scheduling. Inverse methods are simpler to develop and require fewer inputs for model identification. They can model changes in energy consumption based on changes in climatic variables and up to a certain extent, occupancy. This makes them easy-to-use and appealing to building managers for evaluating any general retrofits, building condition monitoring, continuous commissioning and short-term load forecasting (STLF). After evaluating several model structures, an elegant model form was derived which can be used to model daily energy consumption; which can be extended to model energy consumption for any specific hour by adding corrective terms. Additionally, adding AR terms to this model makes it usable for STLF. Two different buildings, one synthetic (ASHRAE medium-office prototype) building and another, an actual office building, were modeled using these techniques. The methodologies proposed have several novel features compared to the manner in which these models have been described earlier. Finally, this thesis investigates characteristic fault signature identification from detailed simulation models and subsequent inverse analysis.
Details
Title
- Leveraging smart meter data through advanced analytics: applications to building energy efficiency
Contributors
- Jalori, Saurabh (Author)
- Reddy, T. Agami (Thesis advisor)
- Bryan, Harvey (Committee member)
- Runger, George C. (Committee member)
- Arizona State University (Publisher)
Date Created
The date the item was original created (prior to any relationship with the ASU Digital Repositories.)
2013
Subjects
Resource Type
Collections this item is in
Note
- thesisPartial requirement for: M.S., Arizona State University, 2013
- bibliographyIncludes bibliographical references (p. 154-159)
- Field of study: Design
Citation and reuse
Statement of Responsibility
by Saurabh Jalori