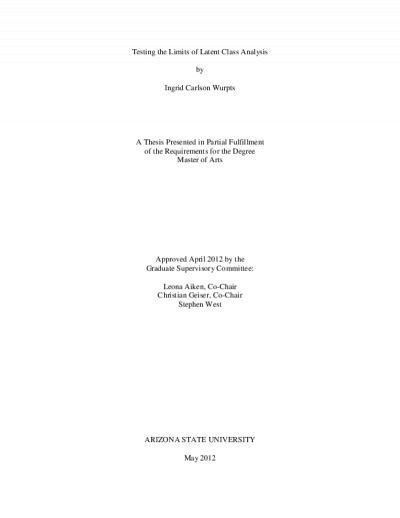
Description
The purpose of this study was to examine under which conditions "good" data characteristics can compensate for "poor" characteristics in Latent Class Analysis (LCA), as well as to set forth guidelines regarding the minimum sample size and ideal number and quality of indicators. In particular, we studied to which extent including a larger number of high quality indicators can compensate for a small sample size in LCA. The results suggest that in general, larger sample size, more indicators, higher quality of indicators, and a larger covariate effect correspond to more converged and proper replications, as well as fewer boundary estimates and less parameter bias. Based on the results, it is not recommended to use LCA with sample sizes lower than N = 100, and to use many high quality indicators and at least one strong covariate when using sample sizes less than N = 500.
Download count: 29
Details
Title
- Testing the limits of latent class analysis
Contributors
- Wurpts, Ingrid Carlson (Author)
- Geiser, Christian (Thesis advisor)
- Aiken, Leona (Thesis advisor)
- West, Stephen (Committee member)
- Arizona State University (Publisher)
Date Created
The date the item was original created (prior to any relationship with the ASU Digital Repositories.)
2012
Subjects
Resource Type
Collections this item is in
Note
-
thesisPartial requirement for: M.A., Arizona State University, 2012
-
bibliographyIncludes bibliographical references (p. 54-56)
-
Field of study: Psychology
Citation and reuse
Statement of Responsibility
by Ingrid Carlson Wurpts